The future of prostate cancer research: bringing data together, looking back and forward
Introduction
Data are key to scientific analysis and progress. The purpose of this article is to provide an opinioned view on current aspects of prostate cancer research with regard to the analysis of digital data. The article has been written from the point of the urologist with a global ambition to improve prostate health. And doing so, it is unavoidable to do that in collaboration with an expert (Henk Obbink) in the field of data acquisition and technology in order to compensate for the urologist (Chris Bangma) lack of deep understanding of data processing and IT developments. This collaboration is far from incidental or symbolical. The authors have been working on various projects during the last 7 years. This article brings together a base for contemplation on how to proceed in this still growing and complex field of scientific development. It stresses the need for a mutual respect and understanding to follow the high ambition through sincere collaboration. Although the medical world appears to be well connected to progress in digitalization, doctors are struggling to bridge their individual gap between scientific developments and day-to-day practice. Without a realistic view on what data can do, expectations are often too high to solve the urgent questions like: which prostatic tumours will become relevant and harmful, which biological pathways related to tumour growth are druggable, which environmental factors need to be influenced to prevent cancer. Therefore, are more data better, and if so which data? How do we obtain these? And how do we analyse those data to give us insight into proper research directions? This article addresses the above challenges, and takes an optimistic view on the effects of global collaborations based on novel information technology.
Data: the new gold
Digitalization of information has initiated an unsurpassed era of analysis in all fields of scientific research during the last thirty years. As computers and information carriers have decreased in price rapidly, the production of digital data has exploded in parallel within a growing number of users, and an increasing capacity of data storage. Genomic analysis of initially DNA, but rapidly afterwards also the various presentations of RNA, has already provided a mountain of information. The size of information appears to become a difficult hurdle to get to the proper understanding of the biologic processes basic to cancer genesis and cancer promotion. And while the good news is that sequencing of genetic materials is getting affordable, almost to a level of application in the daily practice (according to Moore’s law the price of sequencing technology halves each year; https://www.genome.gov/27541954/dna-sequencing-costs-data), this increases the amount of information rapidly. Which means that the bad news is: there is a delay in understanding how to make sense out of this pile of data, in order to make it support health management. Of course, it is not only genomic information, but in the field of urology and especially prostate cancer also digital pathology, imaging, longitudinal clinical follow-up, and other omics on proteins, metabolism, and even lifestyle are increasingly relevant and adding to the pile.
Those who have already digitalized their information in their systems do well in terms of quality control, outcome analysis, and basic science publications. Digitalized data can be mobilized relatively easy and fast, and simple analyses can be performed to show statistical relations over time. For clinicians the longitudinal data on outcome of screening or treatments (prostatectomy and radiotherapy) have provided a wealth of insight on tumor prognosis and stratification of risks. In combination with data from multiple partners important landmark papers can be produced that are altering medical practice (1). Looking at the results of data analyses of the screening consortium ERSPC, we see over the years a steady production of well cited clinically relevant papers (www.erspc.org). For preclinical research a continuous stream of publications on biological molecular data has created great expectations for even more important game changers, like drugs for personalized medicine (2), or tumor prevention (3). But only few of those published results so far have a direct impact in daily practice on the national health policy level. This might be due to the fact that discoveries in this scientific area are still relatively young, but also to the lack of validation studies for disease interventions that may take long to monitor relevant outcomes, and therefore are costly when related to the economic impact and gain they are expected to have. For example: life style changes that are expected to alter methylation status of tumours need many study participants, are difficult to monitor genomically, and take many years to deliver results. Nevertheless, institutes that own biobanks based on clinically well annotated materials in which potential proteomic or genomic biomarkers can be discovered and validated have successfully produced reports and publications for short term effects (4). So, many factors contribute to the slow development and the lack of clinical success in this field, and are related to the difficulties around validation of laboratory findings, valorization, and financing, to mention a few. Therefore, we might ask ourselves which expectations on the results of analysis of data are realistic, and which limitations exist on the methods currently in use.
The nature of data analysis has changed: fishing
Nowadays, basic research activities appear to concentrate on extracting testable hypotheses out of meaningless data from large information sets. While traditionally clinical observations led to ideas that were used to define a study question, now the fishing of statistical relations between invisible phenomena often is the start of further action. The bioinformatics programs used for that are just as obscure to understand by the average urologist as is the working of their own brain. So, replacing the one with the other is one change, but moving towards the use of abstract ‘data’ is another. These data, derived from complex biological processes, usually show the same variability as clinical observations: they depend heavily on the technique that was used to produce them, they vary over time (instable), and relate to an unknown number of confounders. Which in the end means: you need a lot of observations in order to get to some level of understanding. So, in order to get to a testable hypothesis, we first need many data, and from there we need to find something that might have impact on the individual. Our creativity to formulate hypotheses is fed by the data haystack in which the predefined computer program already started to find the needle. For example: at the moment it was understood that looking differently at the genomic data delivered the finding of relevant fusion genes in prostate cancer, we started to find many of these, and appreciated their impact on the disease mechanism, as well as the genomic heterogeneity of the disease (5).
The technology: towards perfection
Data storage and mobilization has become a profession that needs an expertise not available with individual physicians. Just like the collection of bio specimens, it requires special conditions and specialized professionals.
Conditions: storage
Data storage requires resources in terabytes (1012 bytes) that are of a number above imagination (more relations than there are molecules in the universe), and data and software specialists on bioinformatics to design the software needed to structure the data to logical outputs. These facilities and expertise are usually not available in health institutes, but can be obtained on line from highly secured hosts and reasonable low cost to cover technological updates and protection. Cloud based systems are improving in security and size. To host the data of 75,000 lung cancer patients, only 40 terabytes are needed, while the monthly upload of Facebook worldwide contains 7 petabytes (1015 bytes).
Human resources: specialists on content and collaboration
Creating meaningful selections and relations between stored data in this enormous mountain of information has become a bio-informatics job that needs to be incorporated in academic organizations and research institutes. Which means that scientific research is likely restricted to (large) academic centers and industries with sufficient resources. Hospitals and urologists have become providers of data, and translators of clinical problems to data analysts in bio-informatics. The analysts need to understand the kind of relations they are asked to look for in order to understand the nature of the data they are working with. For example: in contrast to age as a quite objective parameter, a lesion found on MRI shows considerable interobserver variability, and the proteome is subject to nocturnal variation. Some urologic departments have enlisted bioinformatics specialists in their research staff in order to keep up with the need to answer questions, to produce scientific output, and to ensure themselves of this analytic support at the time they want (which often is a daily request…).
The outsourcing of data storage and bio-informatics has grown far beyond institutional capacity, so data firms are hired to solve this request, filling the gap of lack of expertise and technology, but creating a different dynamic of dependencies. The resulting physical distance between collaborators might influence the level of mutual trust and understanding. In extreme cases, together with the knowledge gap between these technical and medical professions, this induces a reduction of motivation to contribute to studies. In practice we often meet with the alienation or even apathy of clinicians when their role has been reduced to producing raw data or biomaterials, affecting the progress of studies negatively.
The new partnership landscape
So, it seems that looking for new biologic relations in large clinical biobanks also has created an enforced need to make new partners. All of the members of: patient groups, urologists, academia, industry, and insurances are part of a puzzle that can only be solved by sincere, effective and coordinated collaboration. The patient providing information or biomaterials for contributing markers is regarded the owner of the data. The ownership, however, is useless unless the individual data are mobilized within a larger group of individuals with adequate technology and standards. The moral obligation within society and the scientific inquisitiveness of researchers brings the potential data to life, while it needs industry to bring specific expertise and create impact in daily practice. The balance is framed by governments and health insurances, that dictate the official playground between parties, and allowing the size of incentives needed to go forward (reimbursements, grants, etc.). Patients claim, rightly so, their influence on the aims and directions of research, but are often restricted by specific knowledge unless advised by professionals. Industries are limited in their actions by the need for (short-term) product application, restricting expensive long-term validation processes. Scientists have an unsatisfied hunger for serendipity. It is not easy to see how to combine these groups, yet they have done so before. Only now the relative contribution to the process of each might have been altered. The traditional role of the physician owning data and expertise has gradually disappeared. Instead, she (the doctor) is guiding the patient through the tons of information he has acquired from the internet. The scientists can do endless discovery in a wealth of unclassified data. The industry will only produce products that are cost efficient, while the government audits the process, and negotiates on the price with the insurance companies.
What do we need?
The goal of current research in the prostate field is on preventing (outgrowth of) cancer to occur, to predict the outcome of interventions early on, and to find new curative or adjuvant interventions (often biologicals such as drugs, but also radiotherapeutic techniques). All together: to modulate the biologic and clinical course of the disease in such a way that symptomatic disease does not occur. It is without discussion that the secondary prevention (screening) of early asymptomatic prostate cancer is successful and can be made efficient, in contrast to screening of many other cancers like pancreatic cancer, or bladder cancer. It is at this early stage that the most benefit might be achieved, for patients as well as for the reduction of health costs (the other stage for costs reduction is at the far end of the disease spectrum, when the application of expensive drugs adds little to quality of life). In contrast to this, the promise of precision medicine (which is: providing a personalised drug for the individual patient) based on genomic analyses of individual cancers has still not been redeemed due to the extreme molecular and genetic heterogeneity of prostate cancers. Precision medicine becomes therefore increasingly precision health: the individual risk assessment based on clinical, familial, social, and environmental factors. The consequence is that we gather all this information and make something clinically relevant from it.
The omics techniques provide information that due to its variability and complexity needs large data sets. These sets might contain unexpected confounders, but also need those in order to raise new hypotheses. These confounders might be host related, such as immunologic diseases, or environmental factors. For example: the composition of our food might provide unknown confounders in the analyses of the data on prostate size over time, but at the same time we would like to have that information registered in order to define its importance. The list of potential confounders in food is nearly endless, difficult to register, and it needs a long observation period in order to become relevant in data analyses. To compensate for these, we might enlarge our dataset with more individuals. This often implies that data has to be extracted from different data sources, or even registries that have not systematically sampled and saved the most relevant date. Therefore, it might be tried to find different and unusual, non-harmonized, data sources, e.g. shopping lists, voluntary registration of eating habits by smart devices, or dinner table pictures. It is difficult to predict whether the analysis of confounders will provide relevant outcomes. Or if it only enhances infobesitas and scientific confusion… The below figure shows the simplified relation between the developments on technology, the potential amount of growing data, and the information that creates individualized risk assessments and treatment choices (precision health) (Figure 1).
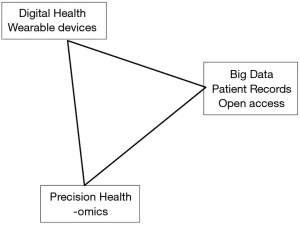
The experience: making larger data sets
The natural reflex on the need of more data is to collaborate with others. Large (inter)national consortia on screening, active surveillance, primary treatment, diagnostics, and metastatic therapy have been established with the expectation that the combination of data is feasible and rewarding. Unless acquired in a prospective standardized way, the data provide an unstructured chaos on unharmonized parameters. There are loads of retrospective data on groups of patients that are unrelated to each other in time and geography. To level that, a large effort needs to be done even to understand the degree of variation between parameters in order to estimate the influence on the data analyses. Is it reasonable to expect that by simply combining already existing clinical data, new insights will be found? Is more of the same data needed from more data centers around the world? Or, alternatively, do we need additional data? There appear to be two options:
- Combining existing data: The ERSPC experience showed that data obtained in different settings in countries all over Europe are difficult to combine. Though the principle of the feasibility and PCa mortality reduction by early detection has been illustrated, a so far unexplained variation in incidence and overdetection remains to be solved. Only by introducing new information on genomics or big data on comorbidity and life style, such problems might be solved. Getting more of the same together, has been tried with the combination of data from alike screening programs, such as the US PLCO trial (6) with ERSPC data. The technical factors needed to combine or compare data sets appeared to be less difficult to solve than the psychological factors that initially led to the competition to publish first. It lacked sincere collaboration during the initial years in which ERSPC and PLCO met on an annual base to compare screening results. The final comparison of the two trials so far only lead to an increased understanding of the different national screening processes and pitfalls, but hardly anything else.
Also, the Movember GAP3 activity belongs to this method of enlarging data sets (7). This global activity supported by the Movember organization builds a database on men with low risk prostate cancer on active surveillance from over 30 institutes from 4 continents. Having included thousands of men with clinical parameters, analysis follows on best practices for selection and monitoring. The first 2 years of the project involved contract negotiations, legal issues, data purification and quality assurance. Creating trust and confidence to bring the data together was a pivotal issue. It remains to be seen how much the combined data will alter current practices, but for sure, men will feel supported when these practices remain identical, now based on the vast amount of high quality global data. More important is that the collaboration serves as an organizational and technical frame to bring other data together on imaging and genomics.
The Movember experience has taught us that the a posteriori integration of the data from experienced institutes requires a lot of extra effort, which might be in the future be avoided when data is collected using the FAIR (Findable, Accessible, Interpretable and Reusable) principles. See (https://www.nature.com/articles/sdata201618). - So, do we need a new source of data beyond the presently available robust clinical information on disease markers, histology, and clinical events? Getting new data on lifestyle is cumbersome. An intense collaboration with patients is needed. The recent sync for science S4S (http://syncfor.science/) initiative might be one of the future enablers. In this US based program started mid 2017 one of the supporters is the government with an explicit role to provide security and privacy of the data. This information contains ten types of data, of which demographics, medications, lab results, vitals, immunizations, smoking, and allergies appear to be easily retrievable by current registries or by wearables. Traditionally patient self-reporting might be instable due to variable reporting compliance, and might lack objectivity. Therefore wearables (technology connected to one’s body reporting continuously or with intervals such as the already commercialized watches (e.g., Philips Health Watch) and access to phones, home computers and registrations (shopping lists, cameras, internet activity) form a rather intrusive source for new objective data. It is difficult to predict whether this will be supported by an unbiased group of volunteering patients. The consumer market indicates an increased use of personal technologies generating data, including genomics. The side effects of data use (the loss of privacy, the commercial use of data, the projected needs for society) are subject of continuous lively dispute. A debate that is covered beyond our pure scientific interest by lawyers, psychologists, politicians… which makes the outcome unpredictable. If we would know where to look for, we might consider bringing more focused data together. But we still have little clue what to pursue.
So far, we have accomplished incidentally the aggregation of data for prostate cancer, indicated by the lower level in Figure 2. We would be ready to access the mid-level of data integration and analysis, at least we think so… Obviously there remains a lot to do for entering the top level to advise individual patients.
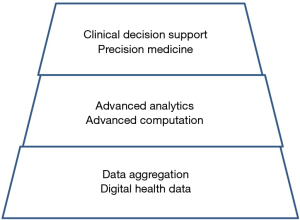
The potential: eliminating PCa
We all want to believe that PCa mortality will be reduced for the majority of men. And not just by the epidemiologic truth that death from other causes competes with that from prostate cancer. We think that new technological developments will provide biologic insights, whether due to the genomic changes in the tumor, or to vascular or immunologic host factors, that will lead to a higher rate of cure. We are able to delay metastases by early screening and treatment. But as men grow older, we need to extend this delay more and more. The race between overall survival and prostate cancer related symptomatic metastases goes on.
As we know from the field described in the articles in this journal, enormous efforts have been necessary to make small steps. To improve on the current level of expertise, even more energy is necessary to make the next steps to proceed. We expect that major changes in imaging and genomics will contribute significantly to prognosis and treatment within the next 5–10 years to come, leading to reduction of over diagnosis and more efficient and affordable therapies. Data analysis is key. But when the success of those analyses turns out to be limited, we might have to add the big data on lifestyle. Which is an enormous challenge with an uncertain outcome.
We have proven that globalization of datasets and sharing is feasible. It requires an ambition that is not satisfied at the level of individual or institutional glamour. The drive for unending improvement starts with accepting collaborating without boundaries in complementary teams. Some people have the opinion that it is easier to collaborate with others when the individual expertise is far diverted, such as between physicians and informaticians. In such cases, little competition occurs, which enhances mutual confidence and sharing research ideas. Sometimes it needs a threat or even a crisis to make the necessary steps to proceed. In Europe it needs the guts to open and share data and materials between the few well organized institutes that harbor good science and good care in the prostate cancer field. Unless these institutes manage to organize themselves in a European consortium of experts, they might not be able to survive as competitive scientists in the long run. Individual institutes will hook up with partners outside Europe in order to survive, and the influence of Europe as an innovative research area declines.
The potential of organizing the data sharing is there. As usual, one of the key elements is defining how the costs can be shared to the benefit of all contributors. While the technological instruments to extract and convert unharmonized data from different individual sources are being designed, the legal instruments still need adaptation. Sharing data and transporting them to a central database will become increasingly challenging with newer European and national regulations on data protection and safety. The use of retrospectively collected biomaterials becomes more and more under strict regulations. Instead sending around research algorithms to the data sources might become part of the solution. Some issues on sharing data and ownership might also be circumvented taking developments in federated databases and block chain technology into account.
Conclusions
Just recently the American College of Cardiology published the 2017 Roadmap for Innovation—ACC Health Policy Statement on Healthcare Transformation in the Era of Digital Health, Big Data, and Precision Health. http://www.onlinejacc.org/content/70/21/2696.full.
Which is a plea for and an example of intensive cooperation among many stakeholders from the cardiology community in order to bring about the necessary transformation of healthcare.
From its summary we quote: “Healthcare transformation is the product of a shared vision between a broad range of stakeholders to establish the future of care delivery and to develop new patient centered, evidence-driven models in which value is rewarded over volume. Important within this transformation are newly developed and rapidly evolving technology-based innovations. These include: digital health with wearable, smartphone, and sensor-based technologies; big data that comprises the aggregation of large quantities of structured and unstructured health information and sophisticated analyses with artificial intelligence, machine learning, and natural language processing techniques; and precision-health approaches to identify individual-level risk and the determinants of wellness and pathogenicity. Although there is promise in the development of such innovations to shift traditional healthcare delivery to virtual and real-time methods and to empower the healthcare enterprise to utilize new technologies and data analytics, there remains a lack of true evaluation of whether these innovations actually improve outcomes and the quality of care. There are major integration challenges across the spectrum of health care for the effective use of new devices, data, and precision-health approaches within existing health information technology systems.”
To chart the future of prostate cancer research a similar initiative is needed and a common roadmap might result which will enable multiple stakeholder to collaborate toward the common goal of eliminating PCa.
As one of the major conclusions from our work we can confirm the African saying: “If you want to go quickly, go alone. If you want to go far, go together”. We would like to add: if you do not want to go, don’t pretend you are going anywhere. So, work on it, or don’t. Make a decision as a group of professionals or scientists.
- In order to perform screening, tumor identification, and targeted therapies better, we need integration of information from imaging, genomics, and biomarkers;
- To integrate (un)structured data better we need block chain technology and knowledgeable analytic people;
- To involve stakeholders convincingly we have to ‘team up’ and provide our common strategy for innovation there where we think it is most needed.
Acknowledgements
None.
Footnote
Conflicts of Interest: The authors have no conflicts of interest to declare.
References
- Auvinen A, Moss SM, Tammela TL, et al. Absolute Effect of Prostate Cancer Screening: Balance of Benefits and Harms by Center within the European Randomized Study of Prostate Cancer Screening. Clin Cancer Res 2016;22:243-9. [Crossref] [PubMed]
- Singh AN, Sharma N. Identification of key pathways and genes with aberrant methylation in prostate cancer using bioinformatics analysis. Onco Targets Ther 2017;10:4925-33. [Crossref] [PubMed]
- Cuzick J, Thorat MA, Andriole G, et al. Prevention and early detection of prostate cancer. Lancet Oncol 2014;15:e484-92. [Crossref] [PubMed]
- Lamy PJ, Allory Y, Gauchez AS, et al. Prognostic Biomarkers Used for Localised Prostate Cancer Management: A Systematic Review. Eur Urol Focus 2017. [Epub ahead of print]. [Crossref] [PubMed]
- Teles Alves I, Hartjes T, McClellan E, et al. Next-generation sequencing reveals novel rare fusion events with functional implication in prostate cancer. Oncogene 2015;34:568-77. [Crossref] [PubMed]
- Tsodikov A, Gulati R, Heijnsdijk EA, et al. Reconciling the Effects of Screening on Prostate Cancer Mortality in the ERSPC and PLCO Trials. Ann Intern Med 2017;167:449-55. [PubMed]
- Bruinsma SM, Bangma CH, Carroll PR, et al. Active surveillance for prostate cancer: a narrative review of clinical guidelines. Nat Rev Urol 2016;13:151-67. [Crossref] [PubMed]