Bioinformatics analysis of the potential regulatory mechanisms of renal fibrosis and the screening and identification of factors related to human renal fibrosis
Introduction
Renal fibrosis is a common pathway to end-stage renal disease (ESRD) during the progression of various chronic kidney diseases (CKDs) (1). Under clinical pathological conditions, due to the shortness of fibrosis increased cytokine expression and regulation, degrading the body occur in renal tubular cell apoptosis, renal tubular interstitial fibroblasts proliferation, leukocyte infiltration, extracellular matrix (ECM) excessively generation and sedimentary features of progressive pathological changes, resulting in a decline in kidney function sex (2-5). In ESRD, renal tissue may present with a loss of normal nephrons, fibroblast proliferation, accumulation of ECM (such as collagen fibers and fibronectin), renal tubulointerstitial fibrosis, and glomerulosclerosis (6).
Considering that kidney biopsy is harmful to the kidney, the application of bioinformatics analysis to identify suitable markers plays an important role in the treatment and prognosis of CKD (7-9). Renal fibrosis is the final pathological outcome of various CKDs and a key factor in the progression of CKD to ESRD. In recent years, multiple mechanisms have been studied during the occurrence of renal fibrosis, including those related to inflammation, macrophage transdifferentiation, and profibrotic pathways (e.g., the abnormal activation of TGF-1/Smad3); however, the exact mechanism remains unclear (10). At present, although blood purification can be used to alleviate the disease, the cost of hemodialysis is high, which results in a heavy economic burden on the families of patients, and hemodialysis can only temporarily delay the condition. Therefore, research into anti-renal fibrosis treatments has become an important issue that is related to national health as well as social and economic development (11-14).
Bioinformatics is an entirely new scientific field that applies computational and analytical tools to capture, analyze, and interpret large amounts of biological data (15). Recently, bioinformatics analysis has been widely used in the life sciences, and high-throughput sequencing can simultaneously provide the expression levels of thousands of genes in each experimental group and directly obtain information on the molecular levels of genes. Moreover, bioinformatics-based microarray and high-throughput sequencing have been widely used to predict potential therapeutic targets for various diseases (16-18). Renal fibrosis is a key pathological change in the formation of ESRD, and is the final pathological outcome of various chronic progressive nephropathy, which seriously endangers human health. In recent years, with the deepening of research, important progress has been made in our understanding of the pathogenesis and potential therapeutic targets of renal fibrosis, but renal fibrosis is still mainly prevented and treated clinically by controlling risk factors that aggravate renal function deterioration, and the prognosis of patients is not significantly improved. In this study, the risk assessment of renal fibrosis was carried out by constructing a prognostic model of renal fibrosis, which has important guiding significance for clinical diagnosis and treatment.
In this study, renal fibrosis chip data were downloaded from the Gene Expression Omnibus (GEO) database, which is an online database of the gene expressions of species, and important targets for regulating renal fibrosis were obtained through dataset analysis (19). We also conducted gene functional enrichment analysis as well as protein-protein interaction (PPI) network and module analysis to further explore the occurrence and development mechanisms of renal fibrosis. The results of this study can provide reliable target genes for basic experiments. We present the following article in accordance with the STREGA reporting checklist (available at https://tau.amegroups.com/article/view/10.21037/tau-22-366/rc).
Methods
Chip analysis of genetic data
Gene expression data were downloaded from the GEO database (https://www.ncbi.nlm.nih.gov/geo/), which is the national biotechnology information of high-throughput gene expression data repository. We selected the GSE102515 dataset, and the platform was GPL13912. The study was conducted in accordance with the Declaration of Helsinki (as revised in 2013).
Screening for differential genes
We analyzed the data and observed the gene expression. We also used the GEO2R online analytical tools to analyze the gene expression differences and filter [filter conditions: P<0.05 and |log2fold change (FC)| >1]. A volcano map was constructed to observe the screened differential genes.
Functional enrichment and pathway enrichment analyses of differential genes
Gene Ontology (GO) analysis is used to annotate genes and gene products and identify biological characteristics. The Kyoto Encyclopedia of Genes and Genomes (KEGG) analysis is a systematic analysis of gene function that relates genomic information to higher-order functional information. The differential genes were annotated using the DAVID database (https://david.ncifcrf.gov/). Gene function enrichment includes molecular function (MF), biological process (BP), and cellular component (CC).
Construction and module analysis of the PPI network
The PPI information in molecular biology was predicted using the STRING database (https://cn.string-db.org/) to search for associations between known and predicted proteins. The differential genes were imported into the STRING database to obtain the interaction data of differential genes. Cytoscape 3.5.0 (Linux, USA) (https://cytoscape.org/) was used to display the differential gene interaction results. The PPI network results were analyzed by module analysis, and the core genes were screened out by the Mcode clustering algorithm related to Cytoscape.
Statistical analysis
Statistical data were analyzed by SPSS 23.0 software (IBM, USA). Quantitative data were represented and independent sample t-tests were employed for comparisons between two groups. One-way analysis of variance (ANOVA) was applied for comparisons between multiple groups, and least significant difference (LSD)-t-test was used for pairwise comparisons between groups. Prism 7 (Graphpad, USA) statistical analysis was applied for target genes, a one-way ANOVA test was used for comparison between groups, and the test level was set at 0.05 (except where otherwise indicated).
Results
Screening for differentially-expressed genes
After quality control of the original data downloaded from the GEO database, a volcano map was drawn (Figure 1). It can be seen that the data quality of all samples is evenly distributed, with the median at about the same level. In addition, the data of each group are closely distributed and there is no overflow value, indicating that the quality of the original data is acceptable.
The gene expression of each sample was mapped into a heat map (Figure 2), indicating that the gene expression trend of each sample in the group was consistent, and the gene expression differences between groups were statistically significant (P<0.05).
Weighted analysis and construction of a central gene network map
The construction of a network system cluster tree using weighted gene co-expression network analysis (WGCNA) was conducted using the dynamic shear method, and gene modules with a height <0.25 were merged. WGCNA is a systems biological method used to describe gene association patterns between different samples, identify highly covarying gene sets, and identify candidate biomarker genes or therapeutic targets based on the interconnectedness of gene sets and the association between gene sets and phenotypes. A total of four gene co-expression modules were obtained after excluding the representatives that failed to be assigned to any known module. Among them, blue modules exhibited the highest correlation with renal fibrosis, and the top 10 genes with the highest connectivity were selected. An interaction network was also drawn was drawn, which indicated that these genes may play an important role in the disease process of fibrosis (Figure 3).
Pathway aggregation of the differentially-expressed genes
The phenotype distribution trend of genes in the correlation table was found by analyzing the signaling pathways of genes with differential expression that were initially screened out. GO is a database established by the Gene Ontology Consortium, which aims to define and describe the functions of genes and proteins applicable to various species. There are three categories in GO database, namely BP, CC, and MF. The possible MFs, CCs, and BPs of gene products are described respectively. These genes were mainly enriched in epithelial cell transdifferentiation and the inflammatory response signaling pathways, and the results were statistically significant (P<0.05). Only a few signaling pathways (Integrin-mediated signaling pathways) were found to be inhibited, suggesting that these genes are primarily involved in mediating inflammatory responses and fibrosis (Figure 4).
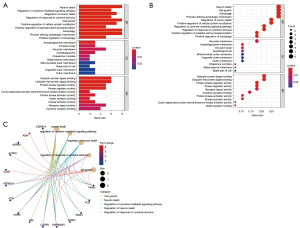
Analysis of immune function status
We used Timer, CIBERSORT, CIBERSORT ABS, and other software to predict the differences in various immune cells in the high- and low-risk groups. The results of most prediction software showed that B cells, CD4+ T cells, CD8+ T cells, myeloid dendrite cells, and other immune cells were different between the high- and low-risk groups. The infiltration integral of immune cells and immune-related functions in both the high- and low-risk groups was quantified by the single-sample gene set enrichment analysis (ssGSEA) algorithm. Correlation analysis showed that immune cell subset (including dendritic cells, B cells, mast cells, neutrophils, helper T cells, and tumor-infiltrating lymphocytes) scores varied between the high- and low-risk groups (P<0.001) (Figure 5).
Discussion
Renal fibrosis is the terminal pathological process of CKD. Clinically, many diseases (such as diabetes, ureteral obstruction, etc.) induce renal fibrosis (20), resulting in excessive deposition of interstitial ECM, scar formation, and fibrosis, which affects the normal function of the kidney and leads to serious impairment of kidney function (21). Therefore, understanding the molecular mechanisms and progression of renal fibrosis is crucial. Renal fibrosis is a common pathway in CKD leading to terminal renal failure. Studies (22-24) have shown that proximal convoluted tubules play an important role in the progression of renal fibrosis, but the molecular mechanism is not completely clear. This study is expected to reveal the molecular mechanism of renal fibrosis induced by proximal convoluted tubules and provide a new target for the prevention and treatment of renal fibrosis.
In this study, we downloaded data from the GEO database and used the GEO2R (https://www.ncbi.nlm.nih.gov/geo/geo2r/) online data analysis tool to divide the data into control and experimental groups. We then searched for differences in gene screening according to P<0.05 and |log2FC| >1 (25). Differential genes were analyzed by GO enrichment. BPs we found to be associated with integrin-mediated signaling pathways. Previous studies (26-28) have shown that integrin alpha V (AV) stimulates the proliferation and activation of renal fibroblasts through TGF signaling, thereby promoting fibrosis (29). The loss of integrin AV has been shown to protect against renal fibrosis in a unilateral ureteral obstruction (UUO), and thus, integrin AV intervention is a key factor in the treatment of CKD characterized by renal fibrosis (30). Also, MF was found to be related to phospholipid binding. Phospholipids include platelet-activating factor (PAF), phosphatidylcholine, and lysophosphatidic acid (LPA). Previous studies (31,32) have shown that PAF induces rat fibroblasts to secrete type I and IV collagen and fibronectin tubular cells, leading to renal fibrosis. Furthermore, LPA exerts therapeutic effects on myoblasts and induces significant expression of connective tissue growth factor. CCs were found to be associated with low-density lipoprotein (LDL) particles, which can aggravate the aggregation of ECM and promote the occurrence and development of glomerular fibrosis by inducing glomerular mesangial cells (MCs).
The selected core genes were ADM, ARRB1, AVPR2, CCR1, MTNR1A, PTH, and S1PR2. ADM significantly alleviates renal fibrosis by inhibiting oxidative stress, gene transfer blocking renal tubular cell inflammation, and inhibiting epithelial-mesenchymal transition (EMT) (33). ADM-induced HO-1 is a potential target for the prevention and treatment of renal fibrosis in patients with CKD. In the ARRB1 family, β-arrestins are involved in various fibrosis-related signal transductions. Interference with Arrestin-1 can significantly improve renal fibrosis and renal injury by inhibiting the Wnt/β-catenin pathway, thus providing a new target for the treatment of renal fibrosis in CKD (34). The AVPR2 arginine vasopressin V2 receptor is a key protein that is required to regulate water permeability in the collecting tube and is mainly involved in the occurrence and development of diabetes insipidus. The CCR1 gene encodes a member of the chemokine receptor family (35). Neutrophils play an important role in mediating fibroblast proliferation, myofibroblast differentiation, stroma formation, and renal tubule atrophy. CCR1, a chemokine receptor that plays a key role in neutrophil recruitment, may be a potential strategy for the treatment of renal fibrosis. Also, MTNR1A melatonin restores the expression level of PRPC in a high glucose state through Akt phosphorylation, thereby preventing the fibrosis induced by high glucose (36). These findings suggest that melatonin may be an effective drug in the treatment of hyperglycemia-induced renal fibrosis. The PTH gene encodes a member of the parathyroid protein family. Previous studies (37,38) have shown that PTH encourages renal fibrosis by promoting the production of TGF-β1 in HK-2 cells. The S1PR2 gene encodes a member of the G-protein-coupled receptor that stimulates additional ECM protein deposition via TGF-β.
Bioinformatics methods were used in this study to analyze the differentially-expressed genes involved in the occurrence of renal fibrosis, and core genes related to renal fibrosis were identified. The results of this study suggest that differentially-expressed genes such as ADM, ARRB1, AVPR2, CCR1, MTNR1A, PTH, and S1PR2 can be used as potential targets for the treatment of renal fibrosis, which provides a direction for further analysis.
Acknowledgments
Funding: None.
Footnote
Reporting Checklist: The authors have completed the STREGA reporting checklist. Available at https://tau.amegroups.com/article/view/10.21037/tau-22-366/rc
Conflicts of Interest: All authors have completed the ICMJE uniform disclosure form (available at https://tau.amegroups.com/article/view/10.21037/tau-22-366/coif). The authors have no conflicts of interest to declare.
Ethical Statement: The authors are accountable for all aspects of the work in ensuring that questions related to the accuracy or integrity of any part of the work are appropriately investigated and resolved. The study was conducted in accordance with the Declaration of Helsinki (as revised in 2013).
Open Access Statement: This is an Open Access article distributed in accordance with the Creative Commons Attribution-NonCommercial-NoDerivs 4.0 International License (CC BY-NC-ND 4.0), which permits the non-commercial replication and distribution of the article with the strict proviso that no changes or edits are made and the original work is properly cited (including links to both the formal publication through the relevant DOI and the license). See: https://creativecommons.org/licenses/by-nc-nd/4.0/.
References
- Gifford CC, Tang J, Costello A, et al. Negative regulators of TGF-β1 signaling in renal fibrosis; pathological mechanisms and novel therapeutic opportunities. Clin Sci (Lond) 2021;135:275-303. [Crossref] [PubMed]
- Yu C, Xiong C, Tang J, et al. Histone demethylase JMJD3 protects against renal fibrosis by suppressing TGFβ and Notch signaling and preserving PTEN expression. Theranostics 2021;11:2706-21. [Crossref] [PubMed]
- Liao Y, Tan RZ, Li JC, et al. Isoliquiritigenin Attenuates UUO-Induced Renal Inflammation and Fibrosis by Inhibiting Mincle/Syk/NF-Kappa B Signaling Pathway. Drug Des Devel Ther 2020;14:1455-68. [Crossref] [PubMed]
- Xu BH, Sheng J, You YK, et al. Deletion of Smad3 prevents renal fibrosis and inflammation in type 2 diabetic nephropathy. Metabolism 2020;103:154013. [Crossref] [PubMed]
- Rayego-Mateos S, Valdivielso JM. New therapeutic targets in chronic kidney disease progression and renal fibrosis. Expert Opin Ther Targets 2020;24:655-70. [Crossref] [PubMed]
- Peng F, Gong W, Li S, et al. circRNA_010383 Acts as a Sponge for miR-135a, and Its Downregulated Expression Contributes to Renal Fibrosis in Diabetic Nephropathy. Diabetes 2021;70:603-15. [Crossref] [PubMed]
- Xu H, Wu T, Huang L. Therapeutic and delivery strategies of phytoconstituents for renal fibrosis. Adv Drug Deliv Rev 2021;177:113911. [Crossref] [PubMed]
- Aranda-Rivera AK, Cruz-Gregorio A, Aparicio-Trejo OE, et al. Redox signaling pathways in unilateral ureteral obstruction (UUO)-induced renal fibrosis. Free Radic Biol Med 2021;172:65-81. [Crossref] [PubMed]
- Wang WJ, Chen XM, Cai GY. Cellular senescence and the senescence-associated secretory phenotype: Potential therapeutic targets for renal fibrosis. Exp Gerontol 2021;151:111403. [Crossref] [PubMed]
- Wei X, Zhu X, Jiang L, et al. Recent advances in understanding the role of hypoxia-inducible factor 1α in renal fibrosis. Int Urol Nephrol 2020;52:1287-95. [Crossref] [PubMed]
- Cohen EP, Olson JD, Tooze JA, et al. Detection and quantification of renal fibrosis by computerized tomography. PLoS One 2020;15:e0228626. [Crossref] [PubMed]
- Wang Y, Wang Y, Xue K, et al. Elevated reactivity of Apelin inhibited renal fibrosis induced by chronic intermittent hypoxia. Arch Biochem Biophys 2021;711:109021. [Crossref] [PubMed]
- Yu YM, Wang W, Wen J, et al. Detection of renal allograft fibrosis with MRI: arterial spin labeling outperforms reduced field-of-view IVIM. Eur Radiol 2021;31:6696-707. [Crossref] [PubMed]
- Liu TT, Luo R, Yang Y, et al. LRG1 Mitigates Renal Interstitial Fibrosis through Alleviating Capillary Rarefaction and Inhibiting Inflammatory and Pro-Fibrotic Cytokines. Am J Nephrol 2021;52:228-38. [Crossref] [PubMed]
- Tian F, Zhang ZY, Sun J, et al. Expression of miR-207 in renal tissue of renal fibrosis rats and its correlation analysis with protein expression of TGF-β1 and Smad3. Eur Rev Med Pharmacol Sci 2021;25:787-94. [PubMed]
- Hreha TN, Collins CA, Daugherty AL, et al. TGFβ1 orchestrates renal fibrosis following Escherichia coli pyelonephritis. Physiol Rep 2020;8:e14401. [Crossref] [PubMed]
- Duan YR, Chen BP, Chen F, et al. LncRNA lnc-ISG20 promotes renal fibrosis in diabetic nephropathy by inducing AKT phosphorylation through miR-486-5p/NFAT5. J Cell Mol Med 2021;25:4922-37. [Crossref] [PubMed]
- Zhou Y, Chai P, Wang J, et al. Wingless/int-1induced secreted protein-1: a new biomarker for renal fibrosis. J Biol Regul Homeost Agents 2021;35:97-103. [PubMed]
- Shen B, Wang F, Zhou Y, et al. Ginsenoside Rh2 inhibits renal fibrosis and renal cell apoptosis in rats with diabetic nephropathy by downregulating discoid domain receptor 1. Nan Fang Yi Ke Da Xue Xue Bao 2021;41:1107-13. [PubMed]
- Chen C, Hou J, Tanner JJ, et al. Bioinformatics Methods for Mass Spectrometry-Based Proteomics Data Analysis. Int J Mol Sci 2020;21:2873. [Crossref] [PubMed]
- Ali MM, Hamid M, Saleem M, et al. Status of Bioinformatics Education in South Asia: Past and Present. Biomed Res Int 2021;2021:5568262. [Crossref] [PubMed]
- Costa MC, Gabriel AF, Enguita FJ. Bioinformatics Research Methodology of Non-coding RNAs in Cardiovascular Diseases. Adv Exp Med Biol 2020;1229:49-64. [Crossref] [PubMed]
- Van Camp PJ, Haslam DB, Porollo A. Bioinformatics Approaches to the Understanding of Molecular Mechanisms in Antimicrobial Resistance. Int J Mol Sci 2020;21:1363. [Crossref] [PubMed]
- Smaïl-Tabbone M, Rance B. Section Editors for the IMIA Yearbook Section on Bioinformatics and Translational Informatics. Contributions from the 2019 Literature on Bioinformatics and Translational Informatics. Yearb Med Inform 2020;29:188-92. [Crossref] [PubMed]
- Rombo SE, Ursino D. Integrative bioinformatics and omics data source interoperability in the next-generation sequencing era-Editorial. Brief Bioinform 2021;22:1-2. [Crossref] [PubMed]
- Wratten L, Wilm A, Göke J. Reproducible, scalable, and shareable analysis pipelines with bioinformatics workflow managers. Nat Methods 2021;18:1161-8. [Crossref] [PubMed]
- Kotulska M, Wojciechowski JW. Bioinformatics Methods in Predicting Amyloid Propensity of Peptides and Proteins. Methods Mol Biol 2022;2340:1-15. [Crossref] [PubMed]
- Orlov YL, Tatarinova TV, Anashkina AA. Bioinformatics Applications to Reveal Molecular Mechanisms of Gene Expression Regulation in Model Organisms. Int J Mol Sci 2021;22:11973. [Crossref] [PubMed]
- Ishack S, Lipner SR. Bioinformatics and immunoinformatics to support COVID-19 vaccine development. J Med Virol 2021;93:5209-11. [Crossref] [PubMed]
- Mohamed SB, Kambal S, Ibrahim SAE, et al. Bioinformatics in Sudan: Status and challenges case study: The National University-Sudan. PLoS Comput Biol 2021;17:e1009462. [Crossref] [PubMed]
- Bai J, Bandla C, Guo J, et al. BioContainers Registry: Searching Bioinformatics and Proteomics Tools, Packages, and Containers. J Proteome Res 2021;20:2056-61. [Crossref] [PubMed]
- Sevigny JL, Norenburg JL, Leasi F. A Bioinformatics Tutorial for Comparative Development Genomics in Diverse Meiofauna. Methods Mol Biol 2021;2219:289-305. [Crossref] [PubMed]
- Sakr MM, Elsayed NS, El-Housseiny GS. Latest updates on SARS-CoV-2 genomic characterization, drug, and vaccine development; a comprehensive bioinformatics review. Microb Pathog 2021;154:104809. [Crossref] [PubMed]
- Meitei NS, Shulaev V. Bioinformatics in Lipidomics: Automating Large-Scale LC-MS-Based Untargeted Lipidomics Profiling with SimLipid Software. Methods Mol Biol 2022;2396:197-214. [Crossref] [PubMed]
- Wang L, Cho J, Satheesh V. Bioinformatics Analysis Guides to LTR Retrotransposon-Derived Extrachromosomal Linear DNAs Identified by ALE-seq. Methods Mol Biol 2021;2250:111-4. [Crossref] [PubMed]
- Cunningham-Oakes E, Trivett H. Applied Bioinformatics and Public Health Microbiology: challenges, discoveries and innovations during a pandemic. Microb Genom 2022;8:000757. [Crossref] [PubMed]
- Gatherer D. Reflections on integrating bioinformatics into the undergraduate curriculum: The Lancaster experience. Biochem Mol Biol Educ 2020;48:118-27. [Crossref] [PubMed]
- Giorgi Silveira R, Perelló Ferrúa C, do Amaral CC, et al. MicroRNAs expressed in neuronal differentiation and their associated pathways: Systematic review and bioinformatics analysis. Brain Res Bull 2020;157:140-8. [Crossref] [PubMed]
(English Language Editor: A. Kassem)