Development and validation of an integrated nomogram to predict personalized new baseline functional outcomes after partial nephrectomy
Introduction
As suggested by recent evidence, the low new baseline glomerular filtration rate (eGFR) after partial nephrectomy (PN) yields a few sequalae with an increased risk of cardiovascular disease and decreased long-term survival (1). In this context, the prediction of personalized new baseline function both before and early after surgery can better contribute to patient counseling and postoperative management planning.
In the field of postoperative renal function management, several variables have been recognized as risk factors for functional deterioration and were integrated so as to comprehensively assess a patient’s specific risk (2-8). However, their instability of testing, only containing preoperative variables, relatively low predictive power, and lack external validation have limited the use of these models. Their advantages are obvious that a patient can better knows his/her specific functional results before surgery. Nevertheless, these flaws can also lead to a decreased accuracy and low reproducibility. As it has been proven that ischemia time (intraoperative variable) and acute kidney injury (AKI) (postoperative variable) are two important factors affecting functional results, but they are not available in these studies (3,6). It is necessary to establish a stable and flexible model integrating pre-, intra-, and post-operative variables to perform better prediction of the new baseline function. In addition, a prediction model established early postoperatively may be able to incorporate more vital variables with higher accuracy, and high-risk patients can receive advanced treatment management early after surgery. To date, no applicable postoperative nomograms integrating pre-, intra- and post-operative variables are available.
The presence of AKI after surgery can predispose patients to chronic kidney disease (1,9). Previous investigations have indicated the severity of AKI with adverse outcomes, and the duration of AKI, which reflects the course of renal functional recovery, is theoretically associated with functional outcomes (9). However, the role of AKI duration after surgery has seldom been investigated in existing studies.
Thus, in this study, we aimed to establish and validate an early postoperative nomogram integrating pre-, intra- and post-operative variables to predict personalized new baseline function in the time frame between 3 and 15 months after PN based on a prospectively maintained institutional database. We aimed specifically at (I) identifying modifiable risk factors so as to prevent worse functional outcomes; (II) whether the incorporating of AKI, ischemia time, and other variables would increase the predictive power; (III) for the first time, integrating AKI duration with other predictors and constructing a comprehensive nomogram for providing simple and precise personalized prediction and high-risk patient prevention. We present the following article in accordance with the TRIPOD reporting checklist (10) (available at https://tau.amegroups.com/article/view/10.21037/tau-21-952/rc).
Methods
The study was conducted in accordance with the Declaration of Helsinki (as revised in 2013). The study was approved by institutional review board of the Army Medical Center Ethical Committee (#2021YiyanLunshen206) and registered in the Chinese Clinical Trial Registry (ChiCTR2100047234). Individual consent for this retrospective analysis was waived.
Workflow and patient selection
The following workflow and patient selection process are illustrated in Figure 1. From January 2014 to December 2017, patients undergoing laparoscopic or robot-assisted PN at a single academic center were retrospectively reviewed in this study. We routinely procedure PN with retroperitoneal approach. Transperitoneal approach is only considered in patients with total anterior location (tumor). The patient inclusion criteria were as follows: (I) age over 18 y/s; (II) completely resected renal tumor; and (III) ipsilateral tumor. The exclusion criteria were as follows: (I) preoperative estimated glomerular filtration rate (eGFR) less than 60 mL/min/1.73 m2 (n=46); (II) solitary kidney (n=9); (III) insufficient renal function data (n=83); and (IV) selective trans-arterial embolization for renal arterial pseudoaneurysm after surgery or radical nephrectomy for recurrence between 3–15 months after surgery (n=11). Additionally, an independent external validation cohort was prospectively collected from January to December 2018 according to the same inclusion and exclusion criteria at the same institution.
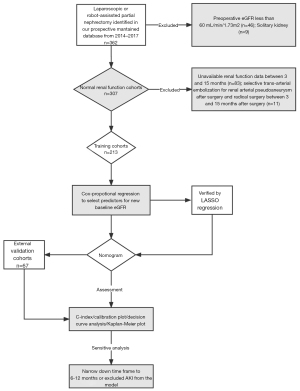
Data collection and definitions
Demographic characteristics and surgical outcomes were extracted from the electronic databases and filled into a predesigned standardized sheet. eGFR was calculated using the Chronic Kidney Disease Epidemiology Collaboration (CKD-EPI) formula. AKI was defined according to the KDIGO criteria and classified into transient AKI and persistent AKI according to a duration less than or over 2 days (48 h) (11) to investigate the role of AKI duration in PN. New baseline function was evaluated in the time frame between 3 and 15 months after PN. In this study, the primary interest was defined as functional deterioration [the eGFR drop percentage over 25% from baseline or the development to CKD 3a or higher (eGFR <60 mL/min/1.73 m2)]. If multiple functional results were recorded between 3 and 15 months after surgery, the one that was the closest to 12 months was collected. Specifically, postoperative and intraoperative variables [in terms of AKI (no vs. yes/no vs. transient vs. persistent) and ischemia time] were incorporated to construct the nomogram. The postoperative landmark was chosen from 3 months, as it was already proven to be a reliable recovery point from surgical insult in a prospective setting (12). The previously reported potential preoperative and postoperative factors were investigated, including age, sex, diabetes, hypertension, baseline eGFR, tumor size, RENAL nephrometry score (RNS), ischemia time, and AKI.
Statistical analysis
First, continuous variables were expressed as the mean ± SD or medians (interquartile ranges), while categorical variables were presented as percent frequency. Second, the new baseline eGFR deterioration, the primary interest, was defined as functional deterioration [the eGFR drop percentage over 25% from baseline or the development to CKD 3a or higher (eGFR <60 mL/min/1.73 m2)]. Third, after verifying the proportional hazard (PH) assumption, a nomogram that predicted functional deterioration between 3 and 15 months after PN was established based on univariate and multivariate Cox regression. Fourth, to prevent overfitting, a new machine learning method, logistic least absolute shrinkage and selection operator (LASSO) regression was utilized to verify the predictors. The LASSO penalty factor (λ) was applied to simultaneously consider contraction and variable selection (13). Fifth, we verified whether AKI duration assisted in improving the predictive power by dividing it into transient or persistent AKI. Sixth, the constructed nomogram was then assessed by discrimination [concordance index (C-index)], calibration [the observed incidence versus the nomogram-predicted probability by the locally weighted scatterplot smoothing (LOWESS) method] and decision curve analysis. The nomogram-derived predictive risk of our endpoint was further divided into a low-risk group and a high-risk group, respectively and Kaplan-Meier analysis was further plotted. Seventh, to test the nomogram sensitivity, we either narrowed down the time frame to 6–12 months or removed AKI from the final model and recalculated the results. Eighth, during the external validation process, the overall point of each patient was calculated based on the nomogram. These points were later incorporated as a factor for Cox regression. The C-index and calibration were derived based on the regression. STATA version 16.1 (Stata Corp., College Station, Tex., USA) was used for data analysis. All tests were two-sided with a significance level set at P<0.05.
Results
The overall demographic data of 213 consecutive patients undergoing robotic-assisted or laparoscopic PN are presented in Table 1. During the time frame between 3 and 15 months after surgery, the average eGFR drop percent was −8.6% (−12.3%, −7.2%), and the mean follow-up period was 8.9 [5–11] months. In addition, AKI was observed in 41 (19.2%) patients, including 20 (9.3%) with transient AKI and 21 (9.9%) with persistent AKI. A total of 40 (18.7%) patients were identified as having eGFR deterioration over 25% during the time frame. Only 9 (4.2%) patients developed 3a CKD or higher.
Table 1
Variables | Training cohorts (n=213) | Validation cohorts (n=67) | P value |
---|---|---|---|
Age (years old) | 54.2±11.7 | 55.4±12.5 | 0.764 |
Female (%) | 90 (42.3) | 29 (41.3) | 0.888 |
Male (%) | 123 (57.7) | 38 (56.7) | |
Hypertension (%) | 58 (27.2) | 15 (22.3) | 0.524 |
Diabetes (%) | 40 (18.7) | 15 (22.3) | 0.477 |
Cardiovascular disease (%) | 45 (21.1) | 16 (23.8) | 0.613 |
RNS | 6.0±1.2 | 6.2±1.1 | 0.818 |
Preoperative eGFR (mL/min/1.73 m2) | 120.1±30.1 | 118.2±39.3 | 0.337 |
60–90 mL/min/1.73 m2 (%) | 27 (12.7) | 5 (7.4) | |
90–120 mL/min/1.73 m2 (%) | 90 (42.3) | 27 (40.2) | |
>120 mL/min/1.73 m2 (%) | 96 (45.0) | 35 (52.2) | |
Tumour diameter (cm) | 3.4±1.4 | 3.7±1.6 | 0.929 |
T stage (%) | |||
1a | 147 (69.0) | 48 (71.6) | 0.117 |
1b | 46 (21.6) | 15 (22.4) | |
2a | 6 (2.8) | 4 (5.9) | |
Unknown | 14 (6.7) | 0 | |
Warm ischemia time (min) | 22.8±7.6 | 21.5±6.9 | 0.106 |
IT over 30 min (%) | 26 (12.2) | 7 (10.4) | 0.829 |
Surgery time (min) | 138±43 | 142±30 | 0.706 |
Blood loss (mL) | 105±52 | 95±50 | 0.084 |
Hospital stay (day) | 6.4±1.9 | 6.2±1.4 | 0.213 |
AKI | |||
No AKI (%) | 172 (80.7) | 55 (82.2) | 0.547 |
Transient AKI (%) | 20 (9.3) | 8 (11.9) | |
Persistent AKI (%) | 21 (9.9) | 4 (5.9) | |
3–15 months eGFR (mL/min/1.73 m2) | 108.8±31.3 | 112.2±29.2 | 0.784 |
<60 mL/min/1.73 m2 (%) | 9 (4.2) | 3 (4.5) | |
60–90 mL/min/1.73 m2 (%) | 55 (25.8) | 14 (20.8) | |
90–120 mL/min/1.73 m2 (%) | 80 (37.5) | 27 (40.3) | |
>120 mL/min/1.73 m2 (%) | 69 (32.4) | 23 (34.2) | |
eGFR drop (%) | −8.6 [−12.3, −7.2] | −8.2 [−12.8, −6.3] | 0.143 |
New baseline functional deterioration (%) | 49 (23.0) | 13 (19.4) | 0.615 |
Follow-up time (month) | 8.9 [5, 11] | 8.2 [5, 12] | 0.892 |
eGFR, estimated glomerular filtration rate; AKI, acute kidney injury; IT, ischemia time.
During the variable selection process, univariate Cox regression identified age (P=0.013), baseline eGFR (P=0.001), RNS (P=0.001), ischemia time (P=0.001), and AKI (P<0.001) as possible risk factors. After the PH (Schönfeld test) test, multivariate Cox regression analyses for new baseline eGFR deterioration were conducted on the risk factors, among which five predictors were identified as statistically significant, including age [hazard ratio (HR) 1.033, 95% confidential interval (CI): (1.007, 1.059), P=0.013], baseline eGFR [HR 1.009, 95% CI: (1.001, 0.017), P=0.048], RNS (HR 1.464, 95% CI: (1.150, 1.863), P=0.002], ischemia time [ischemia time >30 min: HR 2.268, 95% CI: (1.168, 4.404) vs. ischemia time <30 min, P=0.016], and AKI [transient AKI: HR 2.209, 95% CI: (1.009, 5.199) vs. no AKI; persistent AKI: HR 4.277, 95% CI: (2.090, 8.752)] (Table 2). In the LASSO regression analysis, we observed that as the penalty factor (λ) increased to the optimal value of 0.019, age, preoperative eGFR, RNS, ischemia time and AKI remained in the model (Figure 2). This result also signified the decisive effect of these factors, which in turn verified the rationale of these predictors for later analysis.
Table 2
Variables | Univariate analysis | Multivariate analysis (model 1) | Multivariate analysis (model 2) | |||||
---|---|---|---|---|---|---|---|---|
HR (95% CI) | P | HR (95% CI) | P | HR (95% CI) | P | |||
Sex | 0.738 (0.415, 1.310) | 0.299 | ||||||
Age | 1.033 (1.007, 1.059) | 0.013* | 1.035 (1.008, 1.062) | 0.010* | 1.033 (1.007, 1.059) | 0.013* | ||
Hypertension | 1.069 (0.599, 1.906) | 0.822 | ||||||
Diabetes | 0.919 (0.501, 1.688) | 0.787 | ||||||
RNS | 1.490 (1.175, 1.890) | 0.001* | 1.418 (1.113, 1.807) | 0.005* | 1.464 (1.150, 1.863) | 0.002* | ||
Baseline eGFR | 1.012 (1.005, 1.019) | 0.001* | 1.009 (1.001, 1.017) | 0.037* | 1.009 (1.001, 1.017) | 0.037* | ||
IT <30 min | 1.000 | 1.000 | 1.000 | |||||
IT >30 min | 2.945 (1.583, 5.480) | 0.001* | 2.326 (1.208, 4.479) | 0.032* | 2.268 (1.168, 4.404) | 0.016* | ||
AKI | ||||||||
No | 1.000 | 1.000 | ||||||
Yes | 5.185 (2.948, 9.118) | <0.001* | 3.208 (1.698, 6.062) | <0.001* | ||||
AKI | ||||||||
No AKI | 1.000 | 1.000 | ||||||
Transient AKI | 3.694 (1.717, 7.945) | 0.001* | 2.209 (1.009, 5.199) | 0.048* | ||||
Persistent AKI | 6.856 (3.591, 13.090) | <0.001* | 4.277 (2.090, 8.752) | <0.001* | ||||
P value from Schönfeld test | 0.324 | 0.373 | ||||||
C-index | 0.884 | 0.910 |
*, P<0.05. AKI, acute kidney injury; C-index, concordance index; CI, confidence interval; eGFR, estimated glomerular filtration rate; HR, hazard ratio; IT, ischemic time; RNS, RENAL Nephrometry Score; Transient AKI, AKI lasts within 2 days (48 h); Persistent AKI, AKI lasts over 2 days (48 h).
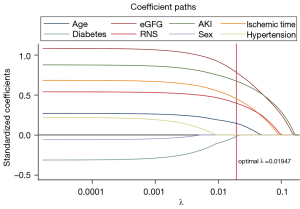
After verification by LASSO regression, the observed probability of the personalized new baseline functional deterioration-free rate was plotted based on the nomogram-predicted probability (Model 2) (Figure 3). Moreover, the C-indices of the two models were 0.884 and 0.910, respectively. The calibration plot of this model was excellent (very close to the 45-degree line) within the whole range of the nomogram-predicted probabilities (Figure 4). The net benefit derived from applying the nomogram in clinical practice, according to the decision curve method, is depicted in Figure 5. The nomogram-derived probability of personalized functional deterioration demonstrated improved clinical risk prediction with threshold probabilities of >5%. Kaplan-Meier analysis was used to plot the significant difference between the low- and high-risk groups (log-rank test: P<0.001, Figure S1) (groups divided by risk tree). Afterward, during the process of external validation based on 67 cases, the C-index was identified to be 0.801 with a good calibration plot and decision curve plot (Figures S2,S3).
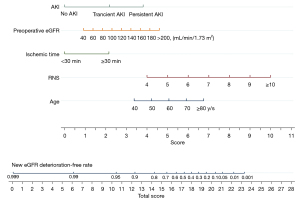
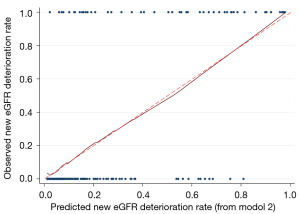
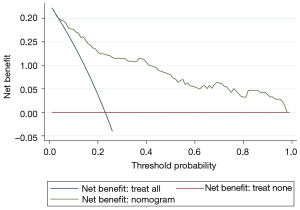
Finally, sensitivity analysis including 184 patients within the time frame of 6–12 months yielded similar results (Table S1). In addition, AKI was excluded from the final model and recalculated, and the remaining four predictors (age, ischemia time, RNS and preoperative eGFR) were still statistically significant (Table S2).
Discussion
The accurate prediction of personalized functional outcomes remains a challenge in the care of patients following PN due to the variants of influencing factors and the complexity of real-world analysis in this personalized medicine era. A large body of evidence supports the concept that three aspects eventually determine the functional outcomes: quality of parenchyma, quantity of preserved parenchyma, and recovery from ischemic insult (14). Nearly all the previous prediction models relied on preoperative characteristics in an attempt at better patient counseling and treatment expectations, but they ignored the point that intraoperative and postoperative factors also played their roles in this field. The existing studies also lacked external validation, and this parameter is crucial for reproducibility in the general population. In the present study, we developed a novel nomogram early postoperatively to predict the personalized new baseline functional deterioration rate. The most related factors were age, preoperative eGFR, ischemic time, RNS, and the presence of AKI and its duration. This nomogram can serve as a stable tool in the daily clinical practice with robust predictive power.
Generally, there are basically two different predictive models in the existing literature. One contains purely radiographic features alone, such as nephrometry scores (5,15,16), while the other contains purely clinical parameters (2,3,6,7). In a review regarding the use and value of nephrometry scores, several scores were identified to examine their correlations with postoperative renal function after meta-analysis (17). However, all these scores are based on radiological imaging. Such inherent characteristics are accompanied by high inconsistency among different practitioners and high complexity, thus limiting their generalizability. By integrating both radiographic and clinical features, the predictive power of our nomogram was enriched.
To the best of our knowledge, there are only a few existing nomograms predicting postoperative renal function and the C-indices vary from 0.70–0.90 (2,3,6,7), which are all lower compared with our model (c-index: 0.910). Similarly, Martini et al. (6) developed a nomogram containing age, sex, Charlson comorbidity index, baseline eGFR, RENAL nephrometry score, AKI in patients with normal baseline renal function, and AKI in patients with CKD. Unexpectedly, ischemic time was not found to be predictors, while our study verified that patients with ischemic time over 30 minutes were 2-times higher possibility of getting functional deterioration. Similar results were obtained in the studies by Abdel Raheem et al. (2), Bertolo et al. (3), and Shum et al. (7). Besides, sex was not found to be a risk factor in our study. Even in the studies mentioned above, either female sex or male sex was an independent risk factor in different studies. This might be due to the differences in baseline features, which should be further investigated in a prospective scenario.
In addition, a slightly higher rate of patients experiencing functional deterioration was observed in our study than in other cohorts (23% vs. ~19%). The most important reason was the difference in the baseline eGFR (mean: 120 vs. ~90 mL/min/1.73 m2). It was proven that a higher preoperative eGFR was associated with a higher probability of eGFR reduction, which was consistent with our findings. Nonetheless, we are confident that the discrepancy will not affect the university of this nomogram because eGFR is a continuous covariate among all studies.
AKI is seldom included in previous studies for analysis, which may be ascribed to its possible interplay with other predictors (6). In our study, we excluded AKI and repeated the analysis, and the remaining four predictors (age, ischemia time, RNS and preoperative eGFR) were still statistically significant (Table S2). In contrast, a model incorporating only age, preoperative eGFR, RNS, and ischemia time demonstrated a lower predictive power (0.794 vs. 0.884, 0.910). Generally, severity and duration are two pillars of AKI, and their value can be enriched in the patient population in trials with functional protection. These two advantages (integrating both radiographic and clinical features; integrating pre-, intra-, and postoperative variables) made our model a lot different from the previous ones and delivered a more robust predictive power.
Some concerns have been raised that AKI may be a time-dependent outcome, since most of the patients presenting with AKI will resolve within days. Under this circumstance, we defined transient and persistent AKI to avoid the time variant, and AKI duration was then proven to be an independent risk factor by the final multivariable Cox regression model. Furthermore, it also improved the predictive power of our model (C-index: 0.910 vs. 0.884).
Our study has several strengths compared with existing studies. For instance, it is the first postoperative applicable predictive model integrating pre-, intra- and post-operative variables, containing both radiographic and clinical features, and it has the value of underlining the importance of AKI duration in contributing to postoperative kidney function during PN functional management. It is one of very few similar nomograms that has been externally validated. In fact, when this is done, a postoperative model can serve as a supplement to previous studies. Furthermore, not only preoperative or postoperative prediction matters but also an ideal prediction system should be dynamic over time. In other words, the prediction should be made pre-, intra- and postoperatively with dynamic management planning. Our study can be a part of this system. In addition, we utilized LASSO regression to verify the predictors before model construction, which made our results more convincing.
Despite the above strengths of this nomogram, several limitations should be noted. First, the retrospective design might miss some less severe patients, while patients with more severe renal function might visit our clinic more frequently and receive more attention during the follow-up period. Nevertheless, we are still confident about our results due to our prospective maintained database. Second, although we included as many risk factors as possible, some other important variables were not collected in our database, such as parenchyma volume, ischemia type, and resection/suturing techniques (18). Third, the nomogram was not externally validated from other centers, which might underestimate the outcome at a non-high-volume center out of their lower technique and management experience (19). Fourth, we did not report oncological outcomes because it was not the interest of this study. Fifth, renal function was evaluated by eGFR, which might not be exact. Sixth, we did not head-to-head compare our model with previous ones because that our intention was to use this nomogram as a supplement not a total replacement. Seventh, the proportion of insufficient renal function data (n=83) is significant even their demographic features are comparable, which may cause selection bias. Besides, patients in our study have relatively small renal tumor with less tumor complexity compared with previous literature.
Notwithstanding its limitations, this novel nomogram we developed in this study may assist in the understanding of personalized strategies in the management of renal surgery and prompt a multidisciplinary evaluation for high-risk patients. In addition, this tool may help patients with high risk (primary low renal function, older age, complicated tumor, high chance of getting long ischemia time or persistent AKI) better balance their choice with oncologic outcomes. This presented study can accurately predict a personalized new baseline eGFR, answering a specific patient’s counseling of how much he/she can get functional deterioration after surgery. As a result, patient with high risk calls for multi-professional consideration and timely management.
Besides the predictive advantages above, our nomogram also carried clinical preventive significance. Compared to age, RNS and preoperative eGFR, ischemia time and AKI are two modifiable risk factors for personalized functional deterioration. Therefore, surgeons should control the ischemia time less than 30 min as possible as he/she can and do everything to avoid AKI (i.e., keep as many healthy parenchymal as possible, maintain liquid balance, avoid using nephrotoxic drugs) to lower a specific patient’s risk. For those with high risk of getting functional deterioration predicted by our nomogram, early multidisciplinary intervention may be needed early after surgery. And the early awareness of functional deterioration for a specific individual can assist him/her to adopt a healthier lifestyle choice.
Acknowledgments
Funding: This study was supported by the Natural Science Foundation of Chongqing (Grant No. cstc2018jcyjAX0666).
Footnote
Reporting Checklist: The authors have completed the TRIPOD reporting checklist. Available at https://tau.amegroups.com/article/view/10.21037/tau-21-952/rc
Data Sharing Statement: Available at https://tau.amegroups.com/article/view/10.21037/tau-21-952/dss
Conflicts of Interest: All authors have completed the ICMJE uniform disclosure form (available at https://tau.amegroups.com/article/view/10.21037/tau-21-952/coif). The authors have no conflicts of interest to declare.
Ethical Statement: The authors are accountable for all aspects of the work in ensuring that questions related to the accuracy or integrity of any part of the work are appropriately investigated and resolved. The study was conducted in accordance with the Declaration of Helsinki (as revised in 2013). The study was approved by institutional review board of the Army Medical Center Ethical Committee (#2021YiyanLunshen206) and registered in the Chinese Clinical Trial Registry (ChiCTR2100047234). Individual consent for this retrospective analysis was waived.
Open Access Statement: This is an Open Access article distributed in accordance with the Creative Commons Attribution-NonCommercial-NoDerivs 4.0 International License (CC BY-NC-ND 4.0), which permits the non-commercial replication and distribution of the article with the strict proviso that no changes or edits are made and the original work is properly cited (including links to both the formal publication through the relevant DOI and the license). See: https://creativecommons.org/licenses/by-nc-nd/4.0/.
References
- Antonelli A, Mari A, Longo N, et al. Role of Clinical and Surgical Factors for the Prediction of Immediate, Early and Late Functional Results, and its Relationship with Cardiovascular Outcome after Partial Nephrectomy: Results from the Prospective Multicenter RECORd 1 Project. J Urol 2018;199:927-32. [Crossref] [PubMed]
- Abdel Raheem A, Shin TY, Chang KD, et al. Yonsei nomogram: A predictive model of new-onset chronic kidney disease after on-clamp partial nephrectomy in patients with T1 renal tumors. Int J Urol 2018;25:690-7. [Crossref] [PubMed]
- Bertolo R, Garisto J, Li J, et al. Development and Internal Validation of a Nomogram for Predicting Renal Function after Partial Nephrectomy. Eur Urol Oncol 2019;2:106-9. [Crossref] [PubMed]
- Bhindi B, Lohse CM, Schulte PJ, et al. Predicting Renal Function Outcomes After Partial and Radical Nephrectomy. Eur Urol 2019;75:766-72. [Crossref] [PubMed]
- Hsieh PF, Wang YD, Huang CP, et al. A Mathematical Method to Calculate Tumor Contact Surface Area: An Effective Parameter to Predict Renal Function after Partial Nephrectomy. J Urol 2016;196:33-40. [Crossref] [PubMed]
- Martini A, Cumarasamy S, Beksac AT, et al. A Nomogram to Predict Significant Estimated Glomerular Filtration Rate Reduction After Robotic Partial Nephrectomy. Eur Urol 2018;74:833-9. [Crossref] [PubMed]
- Shum CF, Bahler CD, Cary C, et al. Preoperative Nomograms for Predicting Renal Function at 1 Year After Partial Nephrectomy. J Endourol 2017;31:711-8. [Crossref] [PubMed]
- Aguilar Palacios D, Wilson B, Ascha M, et al. New Baseline Renal Function after Radical or Partial Nephrectomy: A Simple and Accurate Predictive Model. J Urol 2021;205:1310-20. [Crossref] [PubMed]
- Bravi CA, Vertosick E, Benfante N, et al. Impact of Acute Kidney Injury and Its Duration on Long-term Renal Function After Partial Nephrectomy. Eur Urol 2019;76:398-403. [Crossref] [PubMed]
- Patzer RE, Kaji AH, Fong Y. TRIPOD Reporting Guidelines for Diagnostic and Prognostic Studies. JAMA Surg 2021;156:675-6. [Crossref] [PubMed]
- Pons B, Lautrette A, Oziel J, et al. Diagnostic accuracy of early urinary index changes in differentiating transient from persistent acute kidney injury in critically ill patients: multicenter cohort study. Crit Care 2013;17:R56. [Crossref] [PubMed]
- Porpiglia F, Fiori C, Bertolo R, et al. Long-term functional evaluation of the treated kidney in a prospective series of patients who underwent laparoscopic partial nephrectomy for small renal tumors. Eur Urol 2012;62:130-5. [Crossref] [PubMed]
- Vasquez MM, Hu C, Roe DJ, et al. Least absolute shrinkage and selection operator type methods for the identification of serum biomarkers of overweight and obesity: simulation and application. BMC Med Res Methodol 2016;16:154. [Crossref] [PubMed]
- Marconi L, Desai MM, Ficarra V, et al. Renal Preservation and Partial Nephrectomy: Patient and Surgical Factors. Eur Urol Focus 2016;2:589-600. [Crossref] [PubMed]
- Kim DK, Jang Y, Lee J, et al. Two-year analysis for predicting renal function and contralateral hypertrophy after robot-assisted partial nephrectomy: A three-dimensional segmentation technology study. Int J Urol 2015;22:1105-11. [Crossref] [PubMed]
- Wang YD, Huang CP, Chang CH, et al. The role of RENAL, PADUA, C-index, CSA nephrometry systems in predicting ipsilateral renal function after partial nephrectomy. BMC Urol 2019;19:72. [Crossref] [PubMed]
- Veccia A, Antonelli A, Uzzo RG, et al. Predictive Value of Nephrometry Scores in Nephron-sparing Surgery: A Systematic Review and Meta-analysis. Eur Urol Focus 2020;6:490-504. [Crossref] [PubMed]
- Porpiglia F, Amparore D, Checcucci E, et al. Parenchymal Mass Preserved after Partial Nephrectomy and "Global Renal Damage": Two Faces of the Same Coin. Eur Urol Oncol 2019;2:104-5. [Crossref] [PubMed]
- Dagenais J, Bertolo R, Garisto J, et al. Variability in Partial Nephrectomy Outcomes: Does Your Surgeon Matter? Eur Urol 2019;75:628-34. [Crossref] [PubMed]