Stemness-associated senescence genes as potential novel risk factors for papillary renal cell carcinoma
Introduction
Renal cell carcinoma (RCC) is the most common cancer affecting the kidney, and 2–3% of adults have this malignancy (1). RCC types can be classified based on their genetic and histological features. Papillary RCC (PRCC) accounts for 10–20% of all RCCs (2); however, data on and evidence about the diagnosis and treatment of PRCC are lacking. The early diagnosis of PRCC is uncommon. Indeed, the majority of patients who are diagnosed with PRCC are already in an advanced stage (3). Surgery is still the primary approach for the treatment of localized diseases; however, up to 40% of surgically resected patients are prone to recurrence or metastasis with a poor prognosis (4).
PRCC is usually classified according to its pathohistological features: a single-layered small cell and scanty cytoplasm are regarded as the signature of type 1 PRCC, while large pseudostratified cells and eosinophilic cytoplasm are regarded as the signature of type 2 PRCC (5). Subtyping is a significant clinical parameter, but the prognosis evaluation value of PRCC morphological subtypes remains controversial. The results of a previous multivariate analysis of 43 PRCC cases revealed that morphological type was associated with PRCC survival (6). However, other studies have reported that the histological subtyping of PRCC has no effect on oncologic outcomes after nephron sparing surgery (7). Thus, prognostic molecular models need to be developed that predict clinical outcomes and contribute to decisions about pharmacotherapy, surgery, and conservative options.
Cancer progression involves transcriptional and epigenetic dysregulation, which frequently leads to oncogenic de-differentiation and the acquisition of stemness features; thus, stemness features indicate greater aggression and resistance to therapy (8). About 6% of RCC cells are regarded as a so-called “side population” that exhibits sphere-forming capacity in vitro and also expresses a set of cancer stem cell markers, including prominin-1 (CD133), paired box gene 2, and Notch Receptor 1. PRCC shares many similarities to proximal tubular progenitor cells, and this type of tumor can develop in a multi-step fashion from benign renal adenomas (9). There were also some researches about the potential novel risk factors for clear cell renal cell carcinoma (ccRCC) (10,11). However, currently, renal carcinoma stem cells are not fully understood, and the putative links between carcinomas and renal stem cells have yet to be elucidated. Focusing on the stemness of PRCC will help to reveal the molecular mechanisms of PRCC initiation and development. Therefore, we used an innovative one-class logistic regression machine learning algorithm (OCLR) to extract transcriptomic and epigenetic feature sets and then obtained the messenger ribonucleic acid stemness index (mRNAsi) for assessing the degree of PRCC stemness based on previous studies (12,13).
Cellular senescence can be described as the stable arrest of the cell cycle coupled with stereotypical phenotypic changes that can contribute to stem cell dysfunction, senescence-related aging phenotypes, chronic diseases, a loss of resilience, and geriatric syndromes (14). Stemness appears to be regulated by senescence via overlapping signaling networks. A previous study showed that the key signaling regulators of the senescence machinery, including p16INK4a, protein 53, p21CIP1 and H3K9me3, operate as critical regulators of stem cell functions (15). Milanovic et al. revealed that senescence evoked cancer cell-intrinsic reprograming into a stem-like state and the acquisition of tumor-initiating potential after their spontaneous escape or forced release from a chemotherapy induced cell-cycle arrest (16). Previous studies have revealed that several gene loci, including tumor suppressor genes, become hypermethylated with age in human and mouse models. The epigenetic defects that accumulate during senescence may specifically affect the function and behavior of stem cells (17). Thus, senescence is not only a fundamental process in PRCC progression, but might provide a mechanistic link between stemness and the malignant progression of PRCC. Based on this, we got aging-related genes from GenAge Database and screened the stemness-associated senescence (SAS) genes by correlation analysis between the expression of aging-related genes in the PRCC samples and their mRNAsi.
We hypothesized that the SAS phenotype of PRCC may confer malignant properties to tumors, resulting in a poor prognosis. Based on this, we sought to clarify the correlation between risk signatures and the abundance of SASs in PRCC.
We present the following article in accordance with the TRIPOD reporting checklist (available at https://dx.doi.org/10.21037/tau-21-913).
Methods
RNA sequence data processing
We obtained the ribonucleic sequencing (RNAseq)-HTSeq-FPKM data of 321 PRCC samples and clinical characteristics of patients with PRCC from the UCSC Xena Database (https://xenabrowser.net/datapages/), which makes comprehensive information and retrieval services accessible from The Cancer Genome Atlas (TCGA) data (18). The clinical features of the PRCC patients are presented in Table 1. Two hundred seventy-one PRCC patients’ clinical data and transcriptome profiles were ultimately obtained. The study was conducted in accordance with the Declaration of Helsinki (as revised in 2013).
Table 1
Characteristics | Patients (n=271) |
---|---|
Age | |
<60 | 108 (39.85%) |
≥60 | 161 (59.41%) |
NA | 2 (0.74%) |
Gender | |
Male | 199 (73.43%) |
Female | 72 (26.57%) |
BMI | |
<18.5 | 5 (1.85%) |
18.5–24.9 | 43 (15.87%) |
>24.9 | 154 (56.83%) |
NA | 69 (25.46%) |
Type | |
I | 72 (26.57%) |
II | 83 (30.63%) |
NA | 116 (42.80%) |
Disease stage | |
I | 170 (62.73%) |
II | 19 (7.01%) |
III | 50 (18.45%) |
IV | 14 (5.17%) |
NA | 20 (7.38%) |
PRCC, papillary renal cell carcinoma; BMI, body mass index; NA, not available.
Calculation of the stemness index
The messenger ribonucleic acid stemness index (mRNAsi) was obtained from previous studies (12,13). Pluripotent stem cell data were obtained from the Progenitor Cell Biology Consortium (PCBC) data set (https://www.synapse.org/#!Synapse:syn1773109/wiki/54962). We then built a predictive model using a one-class logistic regression (OCLR) algorithm based on the data set. The workflow can be found at https://bioinformaticsfmrp.github.io/PanCanStem_Web/. We used the stem index model to score PRCC samples from TCGA data sets.
Screening SAS genes
Aging-related genes were accessed via the GenAge Database (available at https://genomics.senescence.info/genes/) (19). A correlation analysis between the expression of aging-related genes in the PRCC samples and their mRNAsi was calculated using Pearson’s correlation coefficients; SAS genes were screened using a P value <0.05.
Identification of the prognostic SAS signature
We conducted a univariate Cox regression model to detect prognostic SASs. SASs with P values <0.05 were identified. These SASs were then analyzed in R statistical software (version 3.5.1) using the “glmnet” package (R Foundation for Statistical Computing, Vienna, Austria) and a least absolute shrinkage and selection operator (LASSO)–Cox regression model analysis was conducted (20). A list of prognostic SAS genes with related coefficients was accessed from the expression profile, and PRCC patients’ overall survival (OS) was determined according to the best lambda value.
Risk score of every PRCC patient based on prognostic SAS signatures
The risk score of each PRCC patient was calculated from the data of the expression level of each prognostic SAS and its corresponding coefficient. For prognostic comparisons, OS was analyzed, and we included 321 PRCC samples with risk scores. We used the “surv_cutpoint” function of the R package “survminer” to obtain the best cut-off points for the continuous variables. The PRCC patients were allocated to the high- and low-risk groups using the best cut-off value of the risk score.
Weighted co-expression network construction and functional enrichment analysis associated with PRCC
A co-expression molecular analysis of the mRNAsi and the risk scores of PRCC patients was conducted using the “WGCNA” package in R (21). To investigate the biological functions of the gene modules and key genes, the Cytoscape plug-in ClueGO (v2.5.2) was used to perform the enrichment analysis based on the Kyoto Encyclopedia of Genes and Genomes (KEGG) and WikiPathways databases. The threshold values were defined as follows: FDR <0.05 and P<0.01. In our study, Cytoscape software was used to visualize the significant functional enrichment results.
Statistical analysis
All statistical analyses were conducted using R (version 3.5.1). The predictive accuracy of the SAS risk score were determined by Kaplan-Meier curves. For Kaplan-Meier curves, P values and hazard ratio (HR) with 95% confidence interval (CI) were generated by log-rank tests and univariate Cox proportional hazards regression. When P value was <0.05, the statistical analyses were defined as statistically significant. Pearson’s chi-square tests were executed for the correlation analysis.
Results
Clinical characteristics of PRCC patients
A flowchart detailing the study’s data preparation, processing, and analysis approach is shown in Figure 1. A total of 271 PRCC patients were enrolled in this study. The detailed clinical characteristics of these patients are summarized in Table 1.
Stemness varies across PRCC and predicts patient survival
We used the stem index model to score the mRNAsi of the PRCC samples (see https://cdn.amegroups.cn/static/public/tau-21-913-1.xlsx). The “Survminer” R package was used to determine the median cut-off point of 0.63 (see Figure 2A). The PRCC patients were then allocated to the mRNAsi high-risk group (n=236) or the mRNAsi low-risk group (n=35). The results of the Cox regression analysis suggested that patients in the high-risk group had lower survival rates than those in the low-risk group (P=0.002; see Figure 2B).
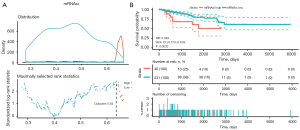
Generation of prognostic SAS genes
We accessed aging-related genes from the GenAge Database. Given the relationship between stemness and aging, we then analyzed aging-related gene expression in PRCC samples. The SAS genes are listed in https://cdn.amegroups.cn/static/public/tau-21-913-2.xlsx (P<0.05, n=295). Using a univariate Cox regression model, we identified a set of 30 prognostic SAS genes (see Figure 3A; P<0.05). A LASSO-Cox regression model was further used to obtain the optimal lambda value from the minimum partial likelihood deviance, which was related to the 8 SAS signatures and was strongly correlated with the prognosis of PRCC patients (see Figure 3B,3C). The expression levels of the 2 SAS signatures of sirtuin-3 (SIRT3) and cyclin-dependent kinase inhibitor 1A (CDKN1A) were revealed by negative coefficients; these 2 SAS signatures were associated with better survival. Conversely, cyclin-dependent kinase 1 (CDK1), heat shock protein family D member 1 (HSPD1), platelet-derived growth factor receptor A (PDGFRA), cyclin-dependent kinase inhibitor 2B (CDKN2B), pyrroline-5-carboxylate reductase 1 (PYCR1), and Sequestosome 1 (SQSTM1) were negatively correlated with better OS. Figure 3D shows the survival status of all the PRCC patients and the heatmap of the 8 SAS signature prognostic genes.
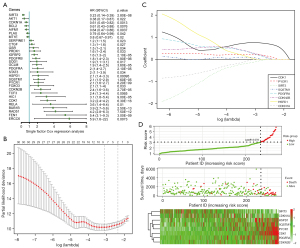
SAS signatures and the patient survival
Based on the expression of these 8 SAS signatures for OS prediction, we established the following formula to calculate the risk score:
Risk score = (0.771443736798139 × CDK1 expression) + (0.296896595577103 × CDKN2B expression) + (0.274905845547291 × PDGFRA expression) + (0.211375135068766 × PYCR1 expression) + (0.0892428222185716 × SQSTM1 expression) + (0.0470091220909639 × HSPD1 expression) + (–0.13579723424102 × SIRT3 expression) + –0.00446166774920266 × CDKN1A expression).
Further, we calculated the SAS signature risk score for every PRCC patient. The patients were partitioned into low- (n=236) and high-risk (n=35) groups based on the median cut-off point of 3.08 (see Figure 4A). The Cox survival analysis showed that being a high-risk group patient was correlated with a lower survival rate [hazards ratio (HR) =0.0502, 95% confidence interval (CI), 0.0253–0.0997, P=1.21e–17; see Figure 4B).
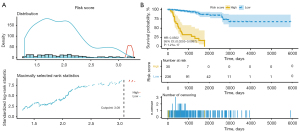
The “Survival ROC” R package was used to generate time-dependent receiver operating characteristic (ROC) curves. Figure 5 shows that the areas under the curve for 1-, 3-, and 5-year OS were 0.949, 0.839, and 0.802, respectively. Given the above, the SAS prognostic signatures performed well in predicting the OS of PRCC patients.
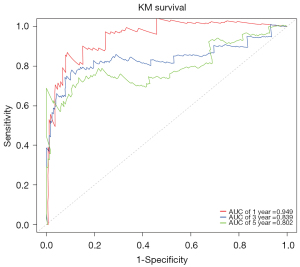
SAS signature association with PRCC type and stage
Next, we examined whether the mRNAsi and risk score were affected by PRCC type or stage. In PRCC, stemness varied markedly across type, such that type 1 had a lower risk score than expected, while type 2 had a higher risk score than expected (see Figure 6A). As for stage, the highest risk score was observed in stage IV and the lowest risk score was observed in stage I (see Figure 6B). Similar to the risk score, the mRNAsi was positively correlated to PRCC type and tumor stage; thus, similar results were found for the mRNAsi (see Figure 6C,6D). In summary, we observed positive correlations between stemness or risk score and PRCC type and tumor stage.
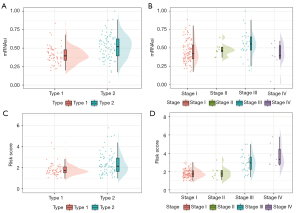
Weighted gene co-expression network analysis and biological pathway identification
The PRCC samples were clustered using Pearson correlation coefficients and an average linkage (default) algorithm (see Figure 7A). We conducted a weighted correlation network analysis (WGCNA) to define molecular co-expression across the mRNAsi and risk scores of PRCC patients and construct the co-expression network. The soft-thresholding parameter (β) was 12, and it was selected that resulted in a scale-free R2 fit of 0.9 (see Figure 7B). Next, 17 modules were identified via average linkage hierarchical clustering—the yellow and green-yellow modules had the highest association with the mRNAsi, while the ink and magenta modules were found to be highly related to risk score (see Figure 7C,7D). These modules were selected for further analysis.
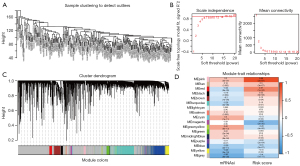
Further, we performed a KEGG enrichment analysis to identify the biological function of the SAS prognostic genes, and found that mRNAsi-related genes were mainly involved in spliceosome, mRNA processing, and the mRNA surveillance pathways (see Figure 8A,8B). Risk score-related genes were mainly involved in the Ataxia Telangiectasia Mutated Protein (ATM) signaling network in the development of disease, base excision repair, and the cell cycle (see Figure 8C,8D).
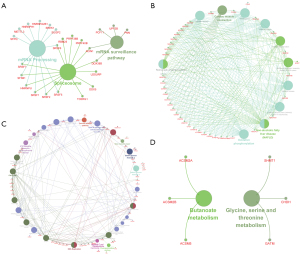
Discussion
PRCC has 2 different subtypes: type 1 and type 2. Type 1 is often related to a higher grade, a later stage, poorer outcomes and a more aggressive disease; however, sensitive and specific indicators have yet to be identified (22,23). Some studies have shown that PRCC histological type is not associated with multifocality, and it seems not to be an argument against conservative surgery (24). Histological type was previously identified as a prognostic factor in a univariate analysis but was not retained in the multivariate analysis (24). Thus, the exploration and establishment of sensitive and reliable diagnostic or typing methods for PRCC is of great importance.
Recent advancements in bioinformatics analyses have raised hopes for the investigation of molecular mechanisms and a wide spectrum of disease predictions. In this study, the risk scores were calculated based on the Cox coefficients of the SAS genes. Kaplan-Meier survival analyses and time-dependent ROCs were used to evaluate SAS genes’ prognostic power. Finally, functional enrichment analyses were performed via molecular co-expression of the mRNAsi and risk scores of the PRCC patients.
The following 8 SAS signatures were found to be highly associated with prognosis in PRCC patients: CDK1, HSPD1, PDGFRA, CDKN2B, PYCR1, SQSTM1, SIRT3, and CDKN1A. Among these SAS signatures, CDK1 is upregulated in PRCC patients and represented the largest proportion of the risk score. Previous study had shown CDK1-related genes could effectively predict the overall survival in a TCGA PRCC cohort (n=287, 741.6 versus 1,623.7 days, P<0.0001) (25). CDK1 drives cell division and there is extensive evidence that CDK1 up-regulation promotes cancer proliferation, migration, and invasion. Our results also showed that the risk scores of related genes were involved in the cell cycle. Additionally, CDK1 is also crucial in controlling stemness. Embryonic stem cells (ESCs) display a particularly high level of CDK1 activity that maintains the epigenetic identity of ESCs. CDK1 phosphorylation modifies many epigenetic regulation proteins and the inhibition of CDK1 phosphorylation could change the histone-modification status of ESCs (26). CDK1 maintains the stemness features of bladder cancers, and potentiates the tumorigenesis and aggressive behavior of bladder cancers via a CDK1-dependent mechanism. Moreover, CDKs activity promotes timely onset of senescence (27). CDK1 stimulates pluripotency and regulates the cell-cycle progression of ESCs by the Thr177‐phosphorylation of TFCP2L1 (28). Consistent with the results of our study, Liu et al. showed that CDK1 was significantly associated with a poor prognosis in lung adenocarcinoma (29).
PYCR1 was also identified as a SAS signature that is upregulated in PRCC patients. Feng et al. confirmed PYCR1 expression had a close relationship with the cancer malignant progression in non-small-cell lung carcinoma, lung cancer, and prostate cancer (30,31). A previous study showed that PYCR1 was over-expressed in PRCC tissues and highly regulated PYCR1 expression led to poor prognosis in PRCC patients (32). PYCR1 has been shown to be related to the aging process. Mutations in the PYCR1 gene cause cutis laxa with progeroid features and mental retardation (33). SIRT3 is localized in mitochondria and plays an important role in mitochondrial biology. The knockout of SIRT3 in nude mice led to the development of several diseases related to aging at an accelerated rate, such as cancer, cardiovascular disease, neurodegenerative disease, and metabolic syndrome (34). In our study, we found that high SIRT3 expression levels in PRCC patients were correlated with better survival. Senescence-associated proliferation arrest is characterized by the activity of the TP53-CDKN1A/p21WAF1 and CDKN2A/p16INK4a-RB pathways (35). In relation to SAS signatures, the results of previous studies and the present study were similar in relation to their basic biological functions, which indicates that we calculated and analyzed an accurate prognostic model for PRCC.
However, our study had several limitations. First, our study only extracted mRNA data from TCGA. The 8 SAS prognostic signatures should be further analyzed by experiments to examine the relationship between their protein expression levels and PRCC stage and type. Second, our study provides evidence that the 8 SAS prognostic signatures were significantly correlated to the survival of PRCC patients; however, the analysis was only conducted via a database; thus, further functional experiments of PRCC need to be conducted. Because of the lack of in vitro and in vivo model in PRCC, the experimental data couldn’t be included in the present study.
Conclusions
In conclusion, we identified 8 SAS prognostic signatures and investigated their prognostic value in PRCC patients. We found that SAS signatures are significantly correlated with stage and type in PRCC. These 8 SAS signatures could be expected to be applied for diagnostic biomarkers for PRCC. Furthermore, the risk scores can be calculated to classify PRCC patients into high- and low-risk groups, and provide better reference values to guide therapy than any single conventional clinical parameter.
Acknowledgments
We would like to thank the administrators of the Open Topic Fund from the Key Laboratory of Clinical Cancer Pharmacology and Toxicology Research of Zhejiang Province (2020E10021).
Funding: This work was supported by the National Natural Science Foundation of China (grant number: 82003853, 82003189), the Zhejiang Provincial Natural Science Foundation (grant numbers: LY20H310001, YY21H310048, and LYQ20H310001), the Medical and Health Research Program of Zhejiang Province (grant numbers: 2018KY297, 2019KY559, 2019KY047, 2022RC008, 2022KY069 and 2021KY040), the “10000 Talents Plan” of Zhejiang Province (to PH), the Zhejiang Provincial Program for the Cultivation of 151 Talents (to PH), the Zhejiang Provincial Program for the Cultivation of High-Level Innovative Health Talents (to PH), and the Zhejiang Provincial Program for the Cultivation of New Heath Talents (to YZ).
Footnote
Reporting Checklist: The authors have completed the TRIPOD reporting checklist. Available at https://dx.doi.org/10.21037/tau-21-913
Conflicts of Interest: All authors have completed the ICMJE uniform disclosure form (available at https://dx.doi.org/10.21037/tau-21-913). YZ received funding from the Zhejiang Provincial Program for the Cultivation of New Heath Talents, the Zhejiang Provincial Natural Science Foundation (LY20H310001), and the Medical and Health Research Program of Zhejiang Province (2021KY040, 2022KY069). YL received funding from the Zhejiang Provincial Natural Science Foundation (LYQ20H310001) and the Medical and Health Research Program of Zhejiang Province (2018KY297, 2022RC008). FS received funding from the National Natural Science Foundation of China (82003853) and the Zhejiang Provincial Natural Science Foundation (YY21H310048). XZ received funding from the National Natural Science Foundation of China (82003189). JS received funding from the Medical and Health Research Program of Zhejiang Province (2019KY047). LL received funding from the Medical and Health Research Program of Zhejiang Province (2019KY559). PH received funding from the “10000 Talents Plan” of Zhejiang Province, the Zhejiang Provincial Program for the Cultivation of 151 Talents, and the Zhejiang Provincial Program for the Cultivation of High-Level Innovative Health Talents. The other authors have no conflicts of interest to declare.
Ethical Statement: The authors are accountable for all aspects of the work in ensuring that questions related to the accuracy or integrity of any part of the work are appropriately investigated and resolved. The study was conducted in accordance with the Declaration of Helsinki (as revised in 2013).
Open Access Statement: This is an Open Access article distributed in accordance with the Creative Commons Attribution-NonCommercial-NoDerivs 4.0 International License (CC BY-NC-ND 4.0), which permits the non-commercial replication and distribution of the article with the strict proviso that no changes or edits are made and the original work is properly cited (including links to both the formal publication through the relevant DOI and the license). See: https://creativecommons.org/licenses/by-nc-nd/4.0/.
References
- Akhtar M, Al-Bozom IA, Al Hussain T. Papillary Renal Cell Carcinoma (PRCC): An Update. Adv Anat Pathol 2019;26:124-32. [Crossref] [PubMed]
- Jiang T, Wu YP, Chen SH, et al. Prognosis and clinicopathological characteristics of renal cell carcinoma: does bilateral occurrence influence overall and cancer-specific survival? Transl Cancer Res 2020;9:432-40. [Crossref]
- Delahunt B, Eble JN, Egevad L, et al. Grading of renal cell carcinoma. Histopathology 2019;74:4-17. [Crossref] [PubMed]
- Yan H, Wei X, Wu A, et al. Nomograms for predicting overall and cancer-specific survival in patients with papillary renal cell carcinoma: a population-based study using SEER database. Transl Androl Urol 2020;9:1146-58. [Crossref] [PubMed]
- Delahunt B, Eble JN. Papillary renal cell carcinoma: a clinicopathologic and immunohistochemical study of 105 tumors. Mod Pathol 1997;10:537-44. [PubMed]
- Allory Y, Ouazana D, Boucher E, et al. Papillary renal cell carcinoma. Prognostic value of morphological subtypes in a clinicopathologic study of 43 cases. Virchows Arch 2003;442:336-42. [Crossref] [PubMed]
- Bigot P, Bernhard JC, Gill IS, et al. The subclassification of papillary renal cell carcinoma does not affect oncological outcomes after nephron sparing surgery. World J Urol 2016;34:347-52. [Crossref] [PubMed]
- Miranda A, Hamilton PT, Zhang AW, et al. Cancer stemness, intratumoral heterogeneity, and immune response across cancers. Proc Natl Acad Sci U S A 2019;116:9020-9. [Crossref] [PubMed]
- Axelson H, Johansson ME. Renal stem cells and their implications for kidney cancer. Semin Cancer Biol 2013;23:56-61. [Crossref] [PubMed]
- Xu WH, Xu Y, Wang J, et al. Prognostic value and immune infiltration of novel signatures in clear cell renal cell carcinoma microenvironment. Aging (Albany NY) 2019;11:6999-7020. [Crossref] [PubMed]
- Zhang C, He H, Hu X, et al. Development and validation of a metastasis-associated prognostic signature based on single-cell RNA-seq in clear cell renal cell carcinoma. Aging (Albany NY) 2019;11:10183-202. [Crossref] [PubMed]
- Malta TM, Sokolov A, Gentles AJ, et al. Machine Learning Identifies Stemness Features Associated with Oncogenic Dedifferentiation. Cell 2018;173:338-54 e15. [Crossref] [PubMed]
- Qin S, Long X, Zhao Q, et al. Co-Expression Network Analysis Identified Genes Associated with Cancer Stem Cell Characteristics in Lung Squamous Cell Carcinoma. Cancer Invest 2020;38:13-22. [Crossref] [PubMed]
- Perez-Mancera PA, Young AR, Narita M. Inside and out: the activities of senescence in cancer. Nat Rev Cancer 2014;14:547-58. [Crossref] [PubMed]
- Zon LI. Intrinsic and extrinsic control of haematopoietic stem-cell self-renewal. Nature 2008;453:306-13. [Crossref] [PubMed]
- Milanovic M, Fan DNY, Belenki D, et al. Senescence-associated reprogramming promotes cancer stemness. Nature 2018;553:96-100. [Crossref] [PubMed]
- Pollina EA, Brunet A. Epigenetic regulation of aging stem cells. Oncogene 2011;30:3105-26. [Crossref] [PubMed]
- Deng M, Bragelmann J, Schultze JL, et al. Web-TCGA: an online platform for integrated analysis of molecular cancer data sets. BMC Bioinformatics 2016;17:72. [Crossref] [PubMed]
- Tacutu R, Thornton D, Johnson E, et al. Human Ageing Genomic Resources: new and updated databases. Nucleic Acids Res 2018;46:D1083-D90. [Crossref] [PubMed]
- Tibshirani R. The lasso method for variable selection in the Cox model. Stat Med 1997;16:385-95. [Crossref] [PubMed]
- Langfelder P, Horvath S. WGCNA: an R package for weighted correlation network analysis. BMC Bioinformatics 2008;9:559. [Crossref] [PubMed]
- Pignot G, Elie C, Conquy S, et al. Survival analysis of 130 patients with papillary renal cell carcinoma: prognostic utility of type 1 and type 2 subclassification. Urology 2007;69:230-5. [Crossref] [PubMed]
- Klatte T, Pantuck AJ, Said JW, et al. Cytogenetic and molecular tumor profiling for type 1 and type 2 papillary renal cell carcinoma. Clin Cancer Res 2009;15:1162-9. [Crossref] [PubMed]
- Mejean A, Hopirtean V, Bazin JP, et al. Prognostic factors for the survival of patients with papillary renal cell carcinoma: meaning of histological typing and multifocality. J Urol 2003;170:764-7. [Crossref] [PubMed]
- Yang CA, Huang HY, Yen JC, et al. Prognostic Value of RNASEH2A-, CDK1-, and CD151-Related Pathway Gene Profiling for Kidney Cancers. Int J Mol Sci 2018;19. [Crossref] [PubMed]
- Michowski W, Chick JM, Chu C, et al. Cdk1 Controls Global Epigenetic Landscape in Embryonic Stem Cells. Mol Cell 2020;78:459-76 e13. [Crossref] [PubMed]
- Mullers E, Silva Cascales H, Burdova K, et al. Residual Cdk1/2 activity after DNA damage promotes senescence. Aging Cell 2017;16:575-84. [Crossref] [PubMed]
- Heo J, Noh BJ, Lee S, et al. Phosphorylation of TFCP2L1 by CDK1 is required for stem cell pluripotency and bladder carcinogenesis. EMBO Mol Med 2020;12:e10880 [Crossref] [PubMed]
- Liu WT, Wang Y, Zhang J, et al. A novel strategy of integrated microarray analysis identifies CENPA, CDK1 and CDC20 as a cluster of diagnostic biomarkers in lung adenocarcinoma. Cancer Lett 2018;425:43-53. [Crossref] [PubMed]
- Zeng T, Zhu L, Liao M, et al. Knockdown of PYCR1 inhibits cell proliferation and colony formation via cell cycle arrest and apoptosis in prostate cancer. Med Oncol 2017;34:27. [Crossref] [PubMed]
- Cai F, Miao Y, Liu C, et al. Pyrroline-5-carboxylate reductase 1 promotes proliferation and inhibits apoptosis in non-small cell lung cancer. Oncol Lett 2018;15:731-40. [PubMed]
- Wang QL, Liu L. PYCR1 is Associated with Papillary Renal Cell Carcinoma Progression. Open Med (Wars) 2019;14:586-92. [Crossref] [PubMed]
- Jin H, Komita M, Koseki H, et al. Sublethal endoplasmic reticulum stress caused by the mutation of immunoglobulin heavy chain-binding protein induces the synthesis of a mitochondrial protein, pyrroline-5-carboxylate reductase 1. Cell Stress Chaperones 2017;22:77-85. [Crossref] [PubMed]
- Chen J, Wang A, Chen Q. SirT3 and p53 Deacetylation in Aging and Cancer. J Cell Physiol 2017;232:2308-11. [Crossref] [PubMed]
- Bernard M, Yang B, Migneault F, et al. Autophagy drives fibroblast senescence through MTORC2 regulation. Autophagy 2020;16:2004-16. [Crossref] [PubMed]
(English Language Editor: L. Huleatt)