Development of a highly sensitive digital PCR assay to quantify long non-coding RNA MYU in urine samples which exhibited great potential as an alternative diagnostic biomarker for prostate cancer
Introduction
Prostate cancer (PCa) is the second leading cause of cancer morbidity in men, accounting for approximately 20% of cancer diagnoses in males (1). The early detection of PCa involves serum prostate specific antigen (PSA) testing and digital rectal examination (DRE), however, the clinical usage of these methods is limited by their major drawbacks. Serum PSA testing lacks specificity for PCa which may lead to misdiagnosis (2,3), and DRE may be a painful and unpleasant experience for patients (4,5). The definitive diagnostic method for PCa is prostate needle biopsy. Following that, pathological sections are analyzed by Gleason score evaluation, which is a widely used histological grading method for PCa. As the Gleason score is significantly associated with the prognosis of PCa, it has been gradually recognized and widely used clinically, and has become an important reference index for the development of PCa treatment plans (6,7). Prostate needle biopsy is an invasive and painful procedure, and men may experience complications such as infection, bleeding, urinary obstruction, and in some cases (8,9), septic shock that results in death (10). Thus, there is an urgent need for new alternatives which are specific and non-invasive, both in the early detection and the clinical definitive diagnosis of PCa.
Urine samples are more convenient and non-invasive compared with blood samples, becoming a promising resource for liquid biopsy (11,12). Previous studies have indicated that several cancer related biomarkers have been successfully detected in urine samples, including mRNAs and long non-coding RNAs (lncRNAs) (13,14). Some of these biomarkers, such as PCA3 and ERG (5,15), were reported to exhibit great potential in predicting PCa. Therefore, urine could be an alternative clinical sample for liquid biopsy to predict PCa.
Accumulating studies demonstrate that lncRNAs, such as SChLAP1 (16), PCA3 (17), PCAT1 (18), MYU (19), PCGEM1, and PRNCR1 (20), have crucial roles in PCa tumorigenesis and metastasis via regulating mRNAs. Among these reported lncRNAs, MYU (ENSG00000261373) has been shown to serve as an oncogene in other cancers, such as colorectal cancer (21). Moreover, it was reported to promote PCa cell proliferation and migration and was significantly upregulated both at the cellular level and at the tumor tissue level (19). The specificity of lncRNA MYU as a biomarker for prostate cancer has been confirmed by cytological experiments (17). Thus, lncRNA MYU may be a new potential diagnostic biomarker for PCa.
Although using urine samples has certain advantages as mentioned above, the mRNA and lncRNA level in urine samples is relatively low due to RNase exposure and low freshness. Therefore, a detection method with a low detection limit is required to analyze the expression levels of mRNAs and lncRNAs in urine samples. The majority of present studies employ real-time quantitative PCR (qPCR) (5,22), which has a relatively high detection limit for the detection of mRNAs and lncRNAs in urine samples. Thus, a more sensitive method is preferred. Digital PCR (dPCR) is known for its more precise (23) and lower detection limit than qPCR (24,25), causing its widespread use, especially in samples with low nucleic acid abundance. Digital PCR is a breakthrough technology that provides ultrasensitive and absolute nucleic acid quantification. It is particularly useful for low-abundance targets, targets in complex backgrounds, copy number variation, rare allele detection (SNPs), and for monitoring subtle changes in target levels that cannot be detected with real-time PCR (23).
In the present study, a method for detecting lncRNA in urine based on digital PCR technology has been constructed, which can be used for non-invasive diagnosis of prostate cancer. Its specificity and sensitivity are higher than PCA as a non-invasive high-sensitivity diagnosis method for prostate cancer. We developed two assays (qPCR and dPCR) to detect the expression level of lncRNA MYU, and compared their performance by evaluating their linearity, analytical sensitivity, repeatability, and reproducibility. We next used the two assays to test the MYU expression level in 100 clinical urine samples, and identified the normalized MYU expression level. Lastly, we evaluated the potential of the normalized MYU expression level as a diagnostic biomarker of PCa by comparing its performance with that of PSA and the Gleason score. Our study presented a potential alternative diagnostic method to predict and evaluate the malignancy of PCa in the clinic. We present the following article in accordance with the STARD reporting checklist (available at https://dx.doi.org/10.21037/tau-21-820).
Methods
Patients
A total of 113 patients were enrolled in this study at The First Affiliated Hospital of Soochow University. However, only 100 patients’ data were analyzed as the results of the house keeping gene measured by dPCR obtained from the rest 13 patients’ urine samples are negative. Among these 100 patients, 59 of them were pathologically diagnosed PCa patients, while the other 41 patients were diagnosed with benign prostatic hyperplasia (BPH) and were chosen as the control group.
The study was approved by the Ethics Committee of The First Affiliated Hospital of Soochow University. All participants provided their written informed consent to participate in this study. The procedures used in this study adhere to the tenets of the Declaration of Helsinki (as revised in 2013).
Construction of the standard recombinant plasmid
In this study, a house keeping gene mRNA, adaptor related protein complex 3 beta 1 subunit (AP3B1), was selected as the control, which has served as a control gene to normalize the expression level of target genes in several previous dPCR studies (26). AP3B1 is a more suitable control for dPCR assays compared with regular controls such as GAPDH and β-actin, as the abundance of AP3B1 in cells is relatively low and can be directly measured by dPCR without further dilutions which may result in an increase in experimental errors. Besides, the relative expression level of AP3B1 in 10 urine samples was measured by qPCR using β-actin as a control. Similar relative expression levels were obtained. Thus, we chose AP3B1 to be the control gene in both the qPCR and dPCR assays we developed in the following experiments.
Cloning the target gene to be amplified into the PET32 vector as a standard plasmid for subsequent amplification by Sangon Biotech. The standard recombinant plasmid was constructed by inserting one copy of lncRNA MYU and one copy of AP3B1 into the pUC57 plasmid. 5×1/10th serial dilutions of the standard recombinant plasmid was employed to evaluate the performance of qPCR and dPCR assays. The original concentration of the standard recombinant plasmid was detected using NanoDrop 2000 (Thermo Scientific) and converted into copy number according to the relative molecular mass of the standard recombinant plasmid.
Sample preparation, RNA extraction, and reverse transcription
About 50 mL urine samples were transferred from the collection cup to a centrifuge tube within 2 hours after donation. Then, the urine sample was centrifuged for 5 min at 4 °C and 3,000 r/min, and the supernatant was discarded to collect the urine sediment which was used to extract total RNA. The total RNA extraction was performed using TRIzol Reagent (Invitrogen). Nanodrop 2000 (Thermo Scientific) was used for both quantification and to check the purity of the total RNA from the urine sediment. Subsequently, the total RNA was reverse transcribed into cDNA using the PrimeScript™ RT reagent kit with gDNA Eraser (TAKARA), which contained a genomic DNA elimination step before the reverse transcription to remove the impact of genomic DNA on the quantification of RNA. Finally, the cDNA was stored at −20 °C before use.
Real time quantitative PCR (qPCR)
qPCR was performed using TB Green® Premix Ex Taq™ II (TAKARA) according to the manufacturer’s instructions. Briefly, a 20-µL mixture including AP3B1 primers or MYU primers (Table 1), the cDNA sample, ROX reference dye II, premix (2X), and H2O was prepared for each reaction. Triplicate reactions were prepared for each cDNA sample. Then, the amplification was performed on the Quant Studio 7 Flex Real-Time PCR System (ABI) and the thermal cycling conditions were: 95 °C 10 min, denaturation 95 °C 15 s, annealing/extension 60 °C 30 s, 40 cycles. A five-point standard curve and a negative control were used in all runs.
Table 1
Primers | Sequence (5'-3') |
---|---|
MYU forward | AGTGGCCGTTTTACAGAGACA |
MYU reverse | CATGCCAAGCTACGGGAAGG |
AP3B1 forward | GATGTTGAAGAGTGGGGGCA |
AP3B1 reverse | TGTGCCAATACAGCTGAGCA |
Digital PCR (dPCR)
dPCR was carried out using Qx200 ddPCR EvaGreen Supermix (Bio-Rad) according to the manufacturer’s instructions. Specifically, a 20-µL mixture containing AP3B1 primers or MYU primers (Table 1), the cDNA sample, premix (2X), and H2O was prepared for each reaction. Triplicate reactions were prepared for each cDNA sample. The 20 µL mixture was loaded into the sample wells of a DG8 Cartridge (Bio-Rad), followed by 70 µL of QX200 Droplet Generation Oil for EvaGreen (Bio-Rad) into the oil wells. Then, the DG8 Cartridge was put into the QX200 Droplet Generator (Bio-Rad) to form droplets which were subsequently transferred into a 96-well PCR plate (Eppendorf). The amplification was performed on the Eppendorf Mastercycler NEXUS (Eppendorf) and the thermal cycling conditions were: 95 °C 5 min, denaturation 95 °C 30 s, annealing/extension 60 °C 1 min, 40 cycles. After the amplification, the plate was put into the QX200 Droplet Reader (Bio-Rad) where the droplets from each well of the plate were read automatically. A five-point standard curve and a negative control were used in all runs.
Statistical analysis
Basic data processing and analysis, such as the calculation of means, medians, standard deviations (SDs), and confidence intervals (CIs), were performed using Microsoft Excel 2010. GraphPad Prism 5 was used to analyze ROC curves and box plots. The linearity of the qPCR and dPCR assays was evaluated using Origin 9.
Results
Performance comparison between the qPCR and dPCR assays
The 10-fold serial dilutions of the standard recombinant plasmid were prepared, and 5 concentrations of the dilutions were selected according to the actual target concentration in samples. They were amplified in triplicates using the dPCR assay and qPCR assay 3 times to compare their performance in linearity, repeatability (intra-assay variability), and reproducibility (inter-assay variability).
The copy numbers of AP3B1 and MYU measured by these two assays were plotted against the corresponding target input copy number and fitted into a linear regression model using Origin 9. According to Figure 1, both dPCR and qPCR showed high R2 values over the target copy number range between 6.7–67,000 copies/reaction. The R2 values of AP3B1 (0.99470) and MYU (0.99484) detected by dPCR were slightly higher than those detected by qPCR (0.99341 and 0.98942, respectively), which might be caused by the poor performance of qPCR in the detection of a low concentration target according to the data in Table 2 (the means of AP3B1 and MYU copy numbers measured by qPCR differed greatly from the actual input copy number).
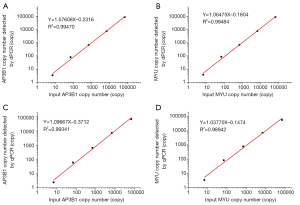
Table 2
Input copy number | dPCR | qPCR | |||||||
---|---|---|---|---|---|---|---|---|---|
Mean | SD | CV% | Mean | SD | CV% | ||||
Measured by AP3B1 copy number | |||||||||
Intra-assay | |||||||||
6.7 | 5.6 | 0.4899 | 0.0875 | 3.7842 | 0.2078 | 0.0549 | |||
67 | 72 | 4.8990 | 0.0680 | 49.0233 | 3.7906 | 0.0773 | |||
670 | 756 | 11.4310 | 0.0151 | 634.3652 | 160.2943 | 0.2527 | |||
6,700 | 7,680 | 130.6395 | 0.0170 | 7,373.0580 | 1,665.7421 | 0.2259 | |||
67,000 | 73,800 | 653.1973 | 0.0089 | 47,713.5150 | 4,396.3400 | 0.0921 | |||
Inter-assay | |||||||||
6.7 | 6.15 | 0.4491 | 0.0730 | 4.4474 | 0.6443 | 0.1449 | |||
67 | 68 | 3.2660 | 0.0480 | 61.1042 | 7.7728 | 0.1272 | |||
670 | 738.5 | 14.2887 | 0.0193 | 592.1821 | 182.4649 | 0.3081 | |||
6,700 | 7,295 | 314.3512 | 0.0431 | 7192.8894 | 612.1393 | 0.0851 | |||
67,000 | 74,900 | 898.1462 | 0.0120 | 54,910.8735 | 6,922.3581 | 0.1261 | |||
Measured by MYU copy number | |||||||||
Intra-assay | |||||||||
6.7 | 6.5 | 1.0614 | 0.1633 | 6.5076 | 2.7492 | 0.4225 | |||
67 | 74.6 | 2.1229 | 0.0285 | 58.8611 | 8.2023 | 0.1394 | |||
670 | 725 | 36.7423 | 0.0507 | 948.4671 | 161.8505 | 0.1706 | |||
6,700 | 7,170 | 269.4439 | 0.0376 | 7,671.9661 | 841.6049 | 0.1097 | |||
67,000 | 72,000 | 816.4966 | 0.0113 | 45,729.6816 | 2,388.0004 | 0.0522 | |||
Inter-assay | |||||||||
6.7 | 6.65 | 0.1225 | 0.0184 | 9.6673 | 1.8473 | 0.1911 | |||
67 | 73.25 | 1.1023 | 0.0150 | 54.9322 | 10.3540 | 0.1885 | |||
670 | 736 | 8.9815 | 0.0122 | 650.8649 | 99.8319 | 0.1534 | |||
6,700 | 7,315 | 118.3920 | 0.0162 | 7,131.5338 | 196.4374 | 0.0275 | |||
67,000 | 75,500 | 2,857.7380 | 0.0379 | 52,931.2508 | 6,786.0819 | 0.1282 |
Table 2 compares the repeatability and reproducibility of dPCR and qPCR. Specifically, the CV values of dPCR in both the intra-assay or inter-assay were lower than the corresponding CV values of qPCR, except for the CV value of dPCR when the input target copy number was 6.7. These data indicated that the repeatability and reproducibility of dPCR were better than those of qPCR.
Evaluation of clinical samples using the qPCR and dPCR assays
Non-DRE urine samples were collected from 100 subjects enrolled between March 21, 2019 and November 5, 2019 who were diagnosed with BPH or PCa. Samples from patients with BPH were selected as controls. For each subject, total RNA was extracted and the copy numbers of AP3B1 and MYU were detected by both the qPCR assay and dPCR assay. A total of 42 out of the 100 CT values of the MYU expression level detected by qPCR were lower than 30 (data not shown), which indicated that the measured MYU expression level might be unreliable. Among these 42 CT values, 13 of them were lower than 35, indicating a negative result of the qPCR assay, while only 3 out of the 100 samples showed a negative result analyzed by dPCR (data not shown). Besides, former data (Figure 1 and Table 2) showed that the performance of dPCR in linearity, repeatability, and reproducibility was better than that of qPCR. Therefore, only data measured by dPCR was analyzed in the subsequent evaluations. The normalized MYU level was calculated through the measured MYU copy number divided by that of AP3B1.
The median age of providers was 71 years and the median serum PSA level was 10.606 ng·mL−1 (Table 3). The serum PSA level was recorded as 100 ng·mL−1 when the clinical data was reported as larger than 100 ng·mL−1, for the convenience of statistics. The median normalized MYU level was 0.3385 copy/1 copy AP3B1. Of all 100 subjects, 59% of them were diagnosed with PCa clinically, and the other 41% were diagnosed with BPH. Among the PCa subjects, the proportion of subjects with a Gleason score of 9 was the highest, accounting for 21% of the total subjects, followed by the percentages of subjects with Gleason scores of 7 and 8, accounting for 17% and 12%, respectively. The proportion of subjects with a Gleason score of 6 was the lowest (6%).
Table 3
Characteristic | Median [range] or % | N; counts/available |
---|---|---|
Age | 71 [43–88] | 100 |
Pre-biopsy serum PSA level (ng·mL–1) | 10.606 [0.247–100] | 100 |
Normalized MYU level (copy/1 copy AP3B1) | 0.3385 [0–3.9304] | 100 |
Positive biopsy result | 59 | 59/100 |
Gleason score | ||
6 | 6 | 6 |
7 | 17 | 17 |
8 | 12 | 12 |
9 | 21 | 21 |
AP3B1, adaptor related protein complex 3 beta 1 subunit.
Evaluation of the performance of PSA and the normalized MYU level as a diagnostic biomarker of PCa
The most frequently used clinical diagnostic biomarker of PCa is the serum PSA level. In the present study, we compared the performance of PSA and the normalized MYU level as a diagnostic biomarker using ROC curve analysis (Figure 2). The AUC of the normalized MYU level (Figure 2B and Table 4) was 0.8475, which was higher than that of PSA (0.8189) (Figure 2A and Table 4). The cutoff values of the serum PSA level and normalized MYU level were 7.781 ng·mL−1 and 0.3112 copy/1 copy AP3B1, respectively (Table 4), which were subsequently used to evaluate their performance in predicting PCa (Figure 3). Compared with the serum PSA level, the normalized MYU level exhibited relatively greater specificity (78.05% vs. 73.17%), NPV (76.19% vs. 75%), and PPV (84.48% vs. 81.67%) for PCa. These results indicated that the normalized MYU level had higher accuracy as a diagnostic biomarker in predicting PCa compared with the serum PSA level.
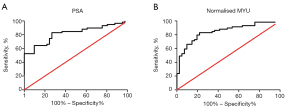
Table 4
Statics index | PSA | MYU |
---|---|---|
AUC | 0.8189 | 0.8475 |
Std. error | 0.04182 | 0.03887 |
95% confidence interval | 0.7369–0.9009 | 0.7713–0.9237 |
P value | <0.0001 | <0.0001 |
Cutoff value | 7.781 | 0.3112 |
ROC, receiver operating characteristic; PSA, prostate specific antigen; AUC, area under the curve.
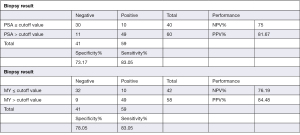
Evaluation of the performance of the normalized MYU level in predicting the prognosis of PCa
The Gleason score is widely used in clinical practice to evaluate the malignancy and predict the prognosis of PCa. ROC curve analysis was carried out to evaluate the relevance between the normalized MYU level and Gleason score (Figure 4A). A statistically significant direct correlation (P=0.0006648<0.001; AUC =0.7694) between the normalized MYU level and Gleason score was observed (Figure 4A). In addition, the box plot (Figure 4B) showed that the median of the normalized MYU level increased according to the increase in Gleason score. Overall, these data indicated that the normalized MYU level showed a direct correlation with Gleason score and could be a potential cofactor to evaluate the malignancy and predict the prognosis of PCa.
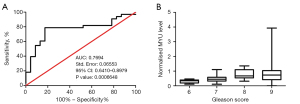
Discussion
In the present study, we developed a highly sensitive dPCR assay to detect MYU and AP3B1 in clinical urine samples. We also analyzed the potential of the normalized MYU level which was shown to be equal to the MYU copy number divided by the AP3B1 copy number in terms of its utility as a diagnostic biomarker in predicting PCa and evaluating the malignancy of PCa. Target RNAs were extracted from non-DRE urine samples in this study, as urine samples are more convenient and non-invasive to obtain compared with blood samples, becoming a promising resource for liquid biopsy (5,27,28). However, the drawbacks of urine samples, such as low freshness and high RNase level, may lead to low yield of lncRNA MYU. Thus, a highly sensitive detection method with a low limit of detection is needed. Previous studies have indicated that dPCR is widely used to detect target genes with a low concentration, such as ctDNA (29), or target genes derived from exosomes (30), FFPE cutaneous biopsies (31), and urine (5,32).
In this study, we employed both qPCR and dPCR to measure lncRNA MYU and compared their performance. Our results demonstrated that only 3 out of 100 samples showed negative results analyzed by the dPCR assay, while 13 samples were out of the detection limit of the qPCR assay. Besides, the dPCR assay exhibited better linearity, repeatability, and reproducibility compared with the qPCR assay (Figure 1 and Table 2). Overall,both two methods of qPCR and dPCR are constructed to measure lncRNA MYU in this study, the results show that dPCR has higher sensitivity and is suitable for the detection of lncRNA MYU with low abundance in urine. A non-invasive prostate cancer screening and prognosis assessment method is constructed, these data indicated that the dPCR assay was more suitable for the detection of lncRNA MYU.
The MYU expression level measured by the dPCR assay was subsequently normalized by a house keeping gene, AP3B1, which has served as a control gene to normalize the expression levels of target genes in several previous dPCR studies (26). The normalization eliminated the experimental errors brought by the differences among the clinical urine samples, such as the variable tumor tissue-derived cell numbers in urinary sediment and the random freshness level of different urine samples, and made it possible to compare the MYU expression level in different samples. Then, the normalized MYU level of urine samples was analyzed to evaluate its performance in predicting PCa by comparing its performance with that of the most frequently used clinical diagnostic biomarker of PCa—PSA. Our results indicated that the normalized MYU level presented relatively greater specificity (78.05% vs. 73.17%), NPV (76.19% vs. 75%), and PPV (84.48% vs. 81.67%) for PCa compared with the serum PSA level (Figure 3). Similarly, a recent study demonstrated that lncRNA MYU was significantly upregulated in PCa when compared with adjacent normal tissues, which also indicated that the MYU expression level could be a potential diagnostic predictor for PCa (19). We evaluated the relevance between the normalized MYU level and the prognosis of PCa by analyzing the correlation between the normalized MYU level and the gold standard approach in clinical practice—the Gleason score. Our data showed a statistically significant direct correlation (P=0.0006648<0.001; AUC =0.7694) between the normalized MYU level and Gleason score (Figure 4A), indicating that there was also a direct correlation between the normalized MYU level and the malignancy of PCa. Meanwhile, MYU has been reported to exert an oncogenic effect in colorectal cancer (21,33) and PCa (19), and promotes PCa cell proliferation and migration through the upregulation of c-Myc expression by sponging miR-184 (19). This indicates that the normalized MYU level did have a direct correlation with the malignancy of PCa, which is in accordance with our results.
Digital PCR provides ultrasensitive and absolute nucleic acid quantification. Its high sensitivity especially be suitable for the detection of low abundance sequences such as lncRNA MYU for prostate cancer. Although this study showed promising results, there were still a few limitations which remain to be discussed. Firstly, one of the three samples which showed a negative result by the dPCR assay was collected from a PCa patient who had bilateral orchiectomy, radioactive particle implantation, and 13 cycles of chemotherapy (the other 2 were BPH patients whose normalized MYU level was supposed to be relatively low). Therefore, this result might be reasonable as these treatments might lead to a reduction in MYU expression since MYU expression presents a direct correlation with the malignancy of PCa. Evaluation of the impact of hormone therapy, chemotherapy, and radiotherapy on the normalized MYU level will be our major focus in future studies. In addition, the data of 13 samples with negative results in terms of the AP3B1 expression level measured by the dPCR assay were excluded from the analysis in this study, which indicated low freshness of these urine samples. Thus, samples will be processed immediately after collection in our future studies.
Conclusions
In summary, we successfully developed a highly sensitive dPCR assay with good linearity, repeatability, and reproducibility, which was especially suitable for detecting MYU and AP3B1 in clinical urine samples. In addition, we identified the novel normalized MYU level which showed promising results as a diagnostic biomarker in predicting and evaluating the malignancy of PCa. To the best of our knowledge, this is the first study to show the crucial role of the expression level of MYU in the development and prognosis of PCa. Moreover, our findings present a non-invasive liquid biopsy method to detect an alternative diagnostic parameter which can assist the diagnosis of PCa in clinical practice.
Acknowledgments
The authors thank Professor Shan Gao and Professor Siyuan Jiang from Suzhou Institute of Biomedical Engineering and Technology for their helpful advice, and Dr. Ru Huang and Dr. Shuai Guo from the First Affiliated Hospital of Soochow University for their assistance with collecting the urine samples.
Funding: This study was funded by National Key R&D Program of China (2017YFF0108600), the Science and Technology Project of Ji Hua Laboratory (X190151TD190), National Major Science and Technology Project of China (2017ZX10302301-003), Instrument Developing Project of the Chinese Academy of Sciences (YJKYYQ20190030), Primary Research & Development Plan of Jiangsu Province (BE2018747, BE2019673), and Science and Technology Project of Suzhou (sys2018101). Key Scientific and Technological Innovation Project of Shandong Province (2019jzy020910).
Footnote
Reporting Checklist: The authors have completed the STARD reporting checklist. Available at https://dx.doi.org/10.21037/tau-21-820
Data Sharing Statement: Available at https://dx.doi.org/10.21037/tau-21-820
Conflicts of Interest: All authors have completed the ICMJE uniform disclosure form (available at https://dx.doi.org/10.21037/tau-21-820). The authors have no conflicts of interest to declare.
Ethical Statement: The authors are accountable for all aspects of the work in ensuring that questions related to the accuracy or integrity of any part of the work are appropriately investigated and resolved. Approval was obtained from the ethics committee of The First Affiliated Hospital of Soochow University. The procedures used in this study adhere to the tenets of the Declaration of Helsinki (as revised in 2013). All participants provided their written informed consent to participate in this study.
Open Access Statement: This is an Open Access article distributed in accordance with the Creative Commons Attribution-NonCommercial-NoDerivs 4.0 International License (CC BY-NC-ND 4.0), which permits the non-commercial replication and distribution of the article with the strict proviso that no changes or edits are made and the original work is properly cited (including links to both the formal publication through the relevant DOI and the license). See: https://creativecommons.org/licenses/by-nc-nd/4.0/.
References
- Barrett T, McLean M, Priest AN, et al. Diagnostic evaluation of magnetization transfer and diffusion kurtosis imaging for prostate cancer detection in a re-biopsy population. Eur Radiol 2018;28:3141-50. [Crossref] [PubMed]
- Loeb S, Vellekoop A, Ahmed HU, et al. Systematic review of complications of prostate biopsy. Eur Urol 2013;64:876-92. [Crossref] [PubMed]
- Milder MTW, Polders DL, Van der Heide UA. PO-0891: Geometrical accuracy of diffusion-weighted magnetic resonance imaging for prostate and rectum. Radiotherapy and Oncology 2014;111:S101. [Crossref]
- Romero FR, Romero AW, Brenny Filho T, et al. Patients' perceptions of pain and discomfort during digital rectal exam for prostate cancer screening. Arch Esp Urol 2008;61:850-4. [Crossref] [PubMed]
- Donovan MJ, Noerholm M, Bentink S, et al. A molecular signature of PCA3 and ERG exosomal RNA from non-DRE urine is predictive of initial prostate biopsy result. Prostate Cancer Prostatic Dis 2015;18:370-5. [Crossref] [PubMed]
- Gottipati S, Warncke J, Vollmer R, et al. Usual and unusual histologic patterns of high Gleason score 8 to 10 adenocarcinoma of the prostate in needle biopsy tissue. Am J Surg Pathol 2012;36:900-7. [Crossref] [PubMed]
- Truesdale MD, Cheetham PJ, Turk AT, et al. Gleason score concordance on biopsy-confirmed prostate cancer: is pathological re-evaluation necessary prior to radical prostatectomy? BJU Int 2011;107:749-54. [Crossref] [PubMed]
- Bjurlin MA, Wysock JS, Taneja SS. Optimization of prostate biopsy: review of technique and complications. Urol Clin North Am 2014;41:299-313. [Crossref] [PubMed]
- Lundström KJ, Drevin L, Carlsson S, et al. Nationwide population based study of infections after transrectal ultrasound guided prostate biopsy. J Urol 2014;192:1116-22. [Crossref] [PubMed]
- Bruyère F, Malavaud S, Bertrand P, et al. Prosbiotate: a multicenter, prospective analysis of infectious complications after prostate biopsy. J Urol 2015;193:145-50. [Crossref] [PubMed]
- Nilsson J, Skog J, Nordstrand A, et al. Prostate cancer-derived urine exosomes: a novel approach to biomarkers for prostate cancer. Br J Cancer 2009;100:1603-7. [Crossref] [PubMed]
- Skog J, Würdinger T, van Rijn S, et al. Glioblastoma microvesicles transport RNA and proteins that promote tumour growth and provide diagnostic biomarkers. Nat Cell Biol 2008;10:1470-6. [Crossref] [PubMed]
- Zheng K, Dou Y, He L, et al. Improved sensitivity and specificity for prostate cancer diagnosis based on the urine PCA3/PSA ratio acquired by sequence-specific RNA capture. Oncol Rep 2015;34:2439-44. [Crossref] [PubMed]
- Tomlins SA, Day JR, Lonigro RJ, et al. Urine TMPRSS2:ERG Plus PCA3 for Individualized Prostate Cancer Risk Assessment. Eur Urol 2016;70:45-53. [Crossref] [PubMed]
- Hendriks RJ, Dijkstra S, Jannink SA, et al. Comparative analysis of prostate cancer specific biomarkers PCA3 and ERG in whole urine, urinary sediments and exosomes. Clin Chem Lab Med 2016;54:483-92. [Crossref] [PubMed]
- Prensner JR, Iyer MK, Sahu A, et al. The long noncoding RNA SChLAP1 promotes aggressive prostate cancer and antagonizes the SWI/SNF complex. Nat Genet 2013;45:1392-8. [Crossref] [PubMed]
- Merola R, Tomao L, Antenucci A, et al. PCA3 in prostate cancer and tumor aggressiveness detection on 407 high-risk patients: a National Cancer Institute experience. J Exp Clin Cancer Res 2015;34:15. [Crossref] [PubMed]
- Prensner JR, Chen W, Han S, et al. The long non-coding RNA PCAT-1 promotes prostate cancer cell proliferation through cMyc. Neoplasia 2014;16:900-8. [Crossref] [PubMed]
- Wang J, Yang X, Li R, et al. Long non-coding RNA MYU promotes prostate cancer proliferation by mediating the miR-184/c-Myc axis. Oncol Rep 2018;40:2814-25. [Crossref] [PubMed]
- Parolia A, Crea F, Xue H, et al. The long non-coding RNA PCGEM1 is regulated by androgen receptor activity in vivo. Mol Cancer 2015;14:46. [Crossref] [PubMed]
- Kawasaki Y, Komiya M, Matsumura K, et al. MYU, a Target lncRNA for Wnt/c-Myc Signaling, Mediates Induction of CDK6 to Promote Cell Cycle Progression. Cell Rep 2016;16:2554-64. [Crossref] [PubMed]
- Frank-Bertoncelj M, Trenkmann M, Kolling C, et al. OP0106 The Impact of Pro-Inflammatory Cytokines on the Expression and Function of Long Noncoding RNA (LNCRNA) in Rheumatoid Arthritis Synovial Fibroblasts. Annals of the Rheumatic Diseases 2014;73:101-101. [Crossref]
- Hindson BJ, Ness KD, Masquelier DA, et al. High-throughput droplet digital PCR system for absolute quantitation of DNA copy number. Anal Chem 2011;83:8604-10. [Crossref] [PubMed]
- Dingle TC, Sedlak RH, Cook L, et al. Tolerance of droplet-digital PCR vs real-time quantitative PCR to inhibitory substances. Clin Chem 2013;59:1670-2. [Crossref] [PubMed]
- Hall Sedlak R, Jerome KR. The potential advantages of digital PCR for clinical virology diagnostics. Expert Rev Mol Diagn 2014;14:501-7. [Crossref] [PubMed]
- Arvia R, Sollai M, Pierucci F, et al. Droplet digital PCR (ddPCR) vs quantitative real-time PCR (qPCR) approach for detection and quantification of Merkel cell polyomavirus (MCPyV) DNA in formalin fixed paraffin embedded (FFPE) cutaneous biopsies. J Virol Methods 2017;246:15-20. [Crossref] [PubMed]
- Miranda KC, Bond DT, Levin JZ, et al. Massively parallel sequencing of human urinary exosome/microvesicle RNA reveals a predominance of non-coding RNA. PLoS One 2014;9:e96094 [Crossref] [PubMed]
- Miranda KC, Bond DT, McKee M, et al. Nucleic acids within urinary exosomes/microvesicles are potential biomarkers for renal disease. Kidney Int 2010;78:191-9. [Crossref] [PubMed]
- Feng WN, Gu WQ, Zhao N, et al. Comparison of the SuperARMS and Droplet Digital PCR for Detecting EGFR Mutation in ctDNA From NSCLC Patients. Transl Oncol 2018;11:542-5. [Crossref] [PubMed]
- Bellingham SA, Shambrook M, Hill AF. Quantitative Analysis of Exosomal miRNA via qPCR and Digital PCR. Methods Mol Biol 2017;1545:55-70. [Crossref] [PubMed]
- Heredia NJ, Belgrader P, Wang S, et al. Droplet Digital™ PCR quantitation of HER2 expression in FFPE breast cancer samples. Methods 2013;59:S20-3. [Crossref] [PubMed]
- Lee H, Park YM, We YM, et al. Evaluation of Digital PCR as a Technique for Monitoring Acute Rejection in Kidney Transplantation. Genomics Inform 2017;15:2-10. [Crossref] [PubMed]
- Yang L, Xu L, Wang Q, et al. Dysregulation of long non-coding RNA profiles in human colorectal cancer and its association with overall survival. Oncol Lett 2016;12:4068-74. [Crossref] [PubMed]