Identification of key genes and microRNA regulatory network in development and progression of urothelial bladder carcinoma
Introduction
Bladder cancer is the ninth most common malignant tumor and about 430,000 new cases occurs per year. Unfortunately, the treatment for bladder cancer is still limited and thus new strategies to obtain clinical benefit are developed. Immunotherapy has been introduced in bladder cancer as early as 1940s by using Bacillus Calmette–Guérin and developed in recent years (1). In preliminary Phase I trial, improved survival rate with 50% response rate was found in bladder cancer patients treated with a monoclonal antibody that targets programmed death-ligand 1 (PD-L1) MPDL3280A (2). Another immunotherapy is that combined Bacillus Calmette–Guérin and IL-10 antibody mediated by Th1 response (3). These immunotherapies hint that changes of tumor microenvironment in bladder cancer is crucial (4,5). Thus, immune factors are valuable as prognostic indicators which still need development and evaluation. On the other hand, immune signatures would promise new combination therapies to improve therapeutic effects for bladder cancer via regulating immune cell infiltration (6).
Besides the tumor microenvironment, large information about pathogeny and markers of bladder cancer are still scarce (7). Fortunately, recent progresses have been made based on multi-omics data and numerous databases facilitate the association studies to identify valuable information (8). For instance, the Cancer Genome Atlas (TCGA) includes over 2.5 petabytes data of multi-omics and corresponding clinical indicators from patients which provides an unprecedented chance to illustrate comprehensive association between cancer development stages and molecular signatures (9). The association in gastric cancer between angiogenesis and cytotoxic signatures were constructed via elucidation by analyzing the data from TCGA which identified key characteristics for potential combination strategy and further clinical investigations (10). Another report using TCGA data to establish association between DNA methylation and gene expression in cancers showing the effects of location of the CpG site in the gene body on gene expression (11). These reports contribute to the understanding of cancer makers for diagnosis and prognosis. However, the global information on correlation between developmental stages of bladder cancer and signatures of transcript expression are still limited. Thus, we presumed that high correlation could be identified which would provide possible markers for aggressive disease and therapeutic information for improved characterization in bladder cancer patients.
In the present study, we performed a direct global single-sample Gene Set Enrichment Analysis (ssGSEA) of gene expression in bladder cancer based from TCGA data. Previous NGS data analysis based on single gene level may missing biological meaningful changes due to gene-level analysis are less obvious than geneset. Biological processes are realized through sets of genes functionally related. By investigating expression alterations on genesets may help to uncover meaningful functional pathways and regulation mechanisms at higher resolutions than single gene levels. Our analyzes indicated that several immunological signatures as well as gene and microRNA (miRNA) expressions were highly correlated with progression of bladder cancer. Furthermore, the characteristics of specific signatures and their prognostic implications were assessed. Collectively, these clues revealed the association of transcript expression signatures and bladder cancer which promise possible prognostic markers. We present the following article in accordance with the STROBE reporting checklist (available at: http://dx.doi.org/10.21037/tau-20-1124).
Methods
Data sources
The RSEM-normalized RNA sequencing data were download from TCGA BLCA Urothelial Bladder Carcinoma (TCGA-BLCA) [https://xenabrowser.net/datapages/?cohort=GDC%20TCGA%20Bladder%20Cancer%20(BLCA)&removeHub=https%3A%2F%2Fxena.treehouse.gi.ucsc.edu%3A443]. In total, 411 samples from urothelial bladder carcinoma patients and 19 samples from adjacent tissues from urothelial bladder carcinoma. Additional data sets including status of patients were downloaded from TCGA database. Genesets data were collected from Molecular Signatures Database (MSigDB) (http://software.broadinstitute.org/gsea/index.jsp, version MSigDB 6.2).
ssGSEA analysis
To build the biological association changes in molecular signaling pathways from urothelial bladder carcinoma patients, the ssGSEA analysis was employed to identify significantly enriched genesets based on TCGA data. The ssGSEA determined genesets in different groups according to functions. The pairwise comparisons were performed in all the experimental conditions and ranked according to changes of expressions. The geneset enrichment score was calculated which represented extent of overrepresentation in each geneset. The ssGSEA analysis was conducted with 1,000 random gene set membership assignments. When P<0.01 and FDR <25%, the genesets were confirmed as significant enrichment. MSigDB was used to identify the gene set definitions as 4 major collections including C2 (curated gene sets), C3 (motif gene sets), C6 (oncogenic gene sets) and C7 (immunologic gene sets). The significant differences between urothelial bladder carcinoma and adjacent tissues were determined by R package: limma with |logFC|>0.01 and adjusted P<0.01.
Determination of association between genesets and phenotypes
To evaluate the association between genesets and phenotypes, we analyzed the survival differences in patients from different clusters including 410 patients from TCGA database. In addition, the tumor stage information from these patients was employed to analyze the correlation between frequency of tumor stages and different clusters.
Effects of genesets on prognosis
The prognosis with expression changes of genesets was assayed using multivariate Cox regression analysis. The multivariate Cox regression analysis was performed from SD top500 geneset by R. P<0.01 as cutoff for significant.
Statistical analysis
All statistical analysis was performed under R version 3.5.3. P<0.01 and FDR <25% were used as cutoffs for significant geneset enrichment outcomes. |logFC| >0.01 and adjusted P<0.01 was considered as statistically significant for differential ssGSEA test between urothelial bladder carcinoma and adjacent tissues. P<0.01 was used as cutoff for significant Cox regression survival analysis.
Results
Correlation between gensets and urothelial bladder carcinoma
We first evaluated the correlation between MSigDB gene sets and urothelial bladder carcinoma incidence by mathematical method, which consisted of 411 cancer tissues and 19 adjacent tissues. The C2 collection includes chemical and genetic perturbations (CGP), canonical pathways (CP), CP:BIOCARTA (BioCarta gene sets), CP:KEGG (KEGG gene sets) and CP:REACTOME (Reactome gene sets) showed that differentiated genesets are mostly cancer related (Figure 1A). In C3 collection, we found that 3 genesets were enriched. Among them, the genes shared upstream cis-regulatory motifs serum response factor (SRF) showed a significant drop in tissues from bladder carcinoma (Figure 1B).
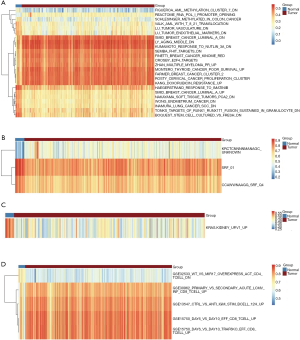
Only 1 gene set as KRAS was enriched in C6 collection. Similar to SRF, the “Genes up-regulated in epithelial kidney cancer cell lines when over-expressing an oncogenic form of KRAS gene” was significantly down regulated in urothelial bladder carcinoma (Figure 1C). Five genesets were determined as enrichments as gene sets related to the immune system in C7 collection. In these genestes, 3 of the 5 genesets were associated with CD8+ T cells showing significant stimulation in carcinoma (Figure 1D).
Association of genesets clusters with bladder cancer
Considered that several genesets from collections of C2, C3, C6 and C7, we used geneset enrichment score to determine crucial genesets clusters which participated in progression of bladder cancer by distance matrix. We used 500 genesets with the largest standard deviation from the four collections. Four clusters were mathematically established and presented in the symmetric heatmaps with pair-wise pearson correlations (Figure 2A). The principal component analysis (PCA) suggested that the 4 clusters could be clearly distinguished (Figure 2B).
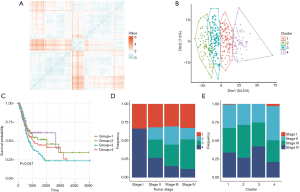
To analyze the survival prognostic probability of 4 clusters, the survival analysis on 410 patients dder carcinoma. The survival analysis proved to be significant (P=0.047). The cluster 3 showed the poorest prognostic while cluster 4 had higher survival prognostic probability (Figure 2C). In addition, the clusters were co-analyzed with tumor histopathological stages. In stage I, only cluster 1 and 4 were found while cluster 2 and cluster 3 were only observed in stage II–IV. Also, we found that the frequency of cluster 4 tended to decrease with tumor stage increase (Figure 2D). The analysis of clusters to tumor stages showed that cluster 3 had the highest frequency in stage IV while cluster 4 had the highest frequency in stage II (Figure 2E).
The heatmap showed the expression significance (adjusted P value <0.05) and the 20 genesets with the largest |log(fold change)| from each cluster. Samples in cluster 3 showed more immune active microenvironments, while samples in cluster 4 exhibited the opposite pattern (Figure 3).
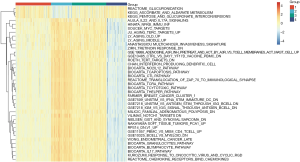
Outcomes with genesets
The cox hazard regression analysis was performed to predict outcomes with genesets and adjusted by age. In C2 collection, activation of Cytosolic Sulfonation and Protease seems prolong the patients’ survival rate (Figure 4A). MiR-450, miR-518s and transcription factor PAX3 appeared as a significant predictor for worse outcome of bladder carcinoma (Figure 4B). In C6 collection, upregulation of KRAS and downregulation of PTEN may predict worse outcomes (Figure 4C). In contrast, active immune microenvironment may predict worse outcomes in C7 collection (Figure 4D).
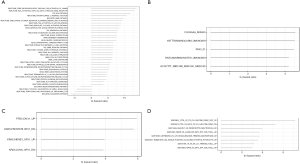
Discussion
A systems biology approach was conducted to build the correlation between MsigDB collection and progression of urothelial bladder carcinoma. This included several genesets that significantly expressed in urothelial bladder carcinoma tissues compared to adjacent tissues, including SRF, KRAS and CD8+ T cells. Lower expression of SRF was unexpected in bladder cancer as present result. Regarding that SRF participates in urinary bladder smooth muscle formation, we proposed that high expression of SRF is attributed to maintaining tissues of bladder (12). CD8+ T cells were significantly activated in bladder cancer deduced from TCGA data. In a previous study, high density of CD8+ T cells were observed and the CD8+ T cells distributed widely in bladder cancer tissues (4,13,14). In addition, the density of CD8+ T cells in bladder cancer had positive correlation with poor prognosis (2). Our result supported that as a marker for prognosis in patients with urothelial bladder carcinoma.
Considering that large amount of genesets that may mediate progression of urothelial bladder carcinoma, we used 500 genesets to determine crucial function cluster. Four clusters were identified and showed different prognosis in patients. This result demonstrated that the method of cluster analysis to determine genesets for cancer progression is a potential approach to reflect global expression patterns of genes mathematically. Similar studies also suggested that analysis of massive data may provide valuable information for understanding molecular basis of cancer development (15-17). Interestingly, the cluster 3 which was highly correlated with the progression had been defined as more immune active microenvironment. Associated with cancer stages, this cluster showed higher frequencies in stage II, III and IV while had the lowest frequency in stage I. Meanwhile, based on our findings, the immune active microenvironment plays a crucial role in stage II and stage III which participates the tumor deterioration especially in invasion and migration. This is similar to previous studies that tumor immune active microenvironment regulates tumor growth and invasion in glioblastoma (18). Barar concluded that tumor microenvironment including immune active microenvironment infiltrates into cancer cells and promotes migration (19). TILs in the tumor immune microenvironment (TIME) have been researched for over 40 years (20). TILs can consist of T, B, and natural killer (NK) cells. CD8+ cytotoxic T-effector cells (TEFF) play a critical role in restraining tumor development, whereas CD4+ Th cells can have pro- or anti-tumor effects (21). TILs have long been proved to be prognosis indicators and predict response to immuno-oncologic treatments (22). Our findings further validated that strategies to treatment on immune active microenvironment influences the success for cancer therapy and understanding of immune subtypes of bladder cancer can help with better personalized treatments.
We then extended the analysis to effect of single geneset on prognosis. In this analysis, we found that miR-450, miR-518s and transcription factor PAX3 in C3 collection. miR-450 associated with human kidney cancer subtypes and cancer cell apoptosis had reported before (23). Overexpression of miR-450a were shown to suppressing multiple genes involved in the epithelial-to-mesenchymal transition (EMT) and reducing tumor migration, invasion and increased anoikis in ovarian tumor cell lines as well as reducing tumor growth in an ovarian tumor xenographic model (24). It has been shown that miR-518a regulated chemokine receptor CCR6 expression in colorectal cancer cells. Low expression of miR-518a-5p was proved to upregulate PIK3C2A and affect the cellular response to the drug, causing resistance to imatinib in GISTs (25). Pax genes consist of a family of transcription factors that are essentially required for the genesis of a variety of tissues and organs. PAX3 is a well-known gene that affects solid tumor alveolar rhabdomyosarcoma (26). Similar to C3 collection, KRAS and PTEN were two factors for prognosis. It is not surprising that upregulation of KRAS had poor outcomes since KRAS is a famous oncogene while downregulation of PTEN was factor for worse outcomes since KRAS is a well-known tumor suppressor gene (27,28). These data suggested that the findings of the present study are similar to major of cancer mechanisms (28-33). Thus, the bioinformatic method for identifying geneset from TCGA data is consistent with result from experimental medicine. But functions of above genes and microRNAs in bladder cancer are still lack of evidence. Our analysis tried to fill the gap and indicated bioinformatic method for identifying geneset from TCGA data is consistent with result from experimental medicine. This approach promises an accuracy and inexpensive method for finding potential markers in cancer. Still, we found that active immune microenvironment had predict worse outcomes in urothelial bladder carcinoma. As found in ssGSEA and cluster analysis, activating immune microenvironment indeed was a signature in bladder cancer. Recently, anti-PD1 immunotherapy is introduced to several cancer and has been proven to be an effective therapy including bladder cancer via regulating immune landscape and gene expressions (34-36). PD-L1 expression had strong associated T-cell infiltration and high expression of genes associated to Th1 and cytotoxic cell function was found in cancers (37-40). Thus, changing the status of immune active microenvironment by anti-PD1 immunotherapy may be a key process.
Conclusions
Our study identified the significant factors infiltrated in urothelial bladder carcinoma. CD8+ T cells, miR-450, miR-518s, transcription factor PAX3, KRAS and PTEN were found highly correlated with urothelial bladder carcinoma as targetable markers. The present data suggested that activating tumor immune microenvironment deteriorated prognosis of patients with bladder cancer. These clues suggested that ameliorate the immune niche may be an effective way for combination therapy. Further experimental and clinical research is required to determine the potential value of controlling tumor immune microenvironment in bladder cancer.
Acknowledgments
Funding: Natural Science Foundation of Hunan Province (2020JJ5915).
Footnote
Reporting Checklist: The authors have completed the STROBE reporting checklist. Available at http://dx.doi.org/10.21037/tau-20-1124
Peer Review File: Available at http://dx.doi.org/10.21037/tau-20-1124
Conflicts of Interest: All authors have completed the ICMJE uniform disclosure form (available at http://dx.doi.org/10.21037/tau-20-1124). The authors have no other conflicts of interest to declare.
Ethical Statement: The authors are accountable for all aspects of the work in ensuring that questions related to the accuracy or integrity of any part of the work are appropriately investigated and resolved.
Open Access Statement: This is an Open Access article distributed in accordance with the Creative Commons Attribution-NonCommercial-NoDerivs 4.0 International License (CC BY-NC-ND 4.0), which permits the non-commercial replication and distribution of the article with the strict proviso that no changes or edits are made and the original work is properly cited (including links to both the formal publication through the relevant DOI and the license). See: https://creativecommons.org/licenses/by-nc-nd/4.0/.
References
- Fuge O, Vasdev N, Allchorne P, et al. Immunotherapy for bladder cancer. Res Rep Urol 2015;7:65-79. [PubMed]
- Powles T, Eder JP, Fine GD, et al. MPDL3280A (anti-PD-L1) treatment leads to clinical activity in metastatic bladder cancer. Nature 2014;515:558-62. [Crossref] [PubMed]
- Böhle A, Brandau S. Immune mechanisms in bacillus Calmette-Guerin immunotherapy for superficial bladder cancer. J Urol 2003;170:964-9. [Crossref] [PubMed]
- Sweis RF, Spranger S, Bao R, et al. Molecular Drivers of the Non-T-cell-Inflamed Tumor Microenvironment in Urothelial Bladder Cancer. Cancer Immunol Res 2016;4:563-8. [Crossref] [PubMed]
- Kahlert C, Kalluri R. Exosomes in tumor microenvironment influence cancer progression and metastasis. J Mol Med (Berl) 2013;91:431-7. [Crossref] [PubMed]
- Kim J, Akbani R, Creighton CJ, et al. Invasive Bladder Cancer: Genomic Insights and Therapeutic Promise. Clin Cancer Res 2015;21:4514-24. [Crossref] [PubMed]
- Martínez-Fernández M, Rubio C, Segovia C, et al. EZH2 in Bladder Cancer, a Promising Therapeutic Target. Int J Mol Sci 2015;16:27107-32. [Crossref] [PubMed]
- Vasaikar SV, Straub P, Wang J, et al. LinkedOmics: analyzing multi-omics data within and across 32 cancer types. Nucleic Acids Res 2018;46:D956-63. [Crossref] [PubMed]
- Tomczak K, Czerwinska P, Wiznerowicz M. The Cancer Genome Atlas (TCGA): an immeasurable source of knowledge. Contemp Oncol (Pozn) 2015;19:A68-77. [Crossref] [PubMed]
- Feng Y, Dai Y, Gong Z, et al. Association between angiogenesis and cytotoxic signatures in the tumor microenvironment of gastric cancer. Onco Targets Ther 2018;11:2725-33. [Crossref] [PubMed]
- Shi Q, Shen L, Gan J, et al. Integrative analysis identifies DNMTs against immune-infiltrating neutrophils and dendritic cells in colorectal cancer. Epigenetics 2019;14:392-404. [Crossref] [PubMed]
- Coletti D, Daou N, Hassani M, et al. Serum Response Factor in Muscle Tissues: From Development to Ageing. Eur J Transl Myol 2016;26:6008. [Crossref] [PubMed]
- Ratliff TL, Ritchey JK, Yuan JJ, et al. T-cell subsets required for intravesical BCG immunotherapy for bladder cancer. J Urol 1993;150:1018-23. [Crossref] [PubMed]
- Peng M, Mo Y, Wang Y, et al. Neoantigen vaccine: an emerging tumor immunotherapy. Mol Cancer 2019;18:128. [Crossref] [PubMed]
- Murdoch TB, Detsky AS. The inevitable application of big data to health care. JAMA 2013;309:1351-2. [Crossref] [PubMed]
- Stephens PJ, Greenman CD, Fu B, et al. Massive genomic rearrangement acquired in a single catastrophic event during cancer development. Cell 2011;144:27-40. [Crossref] [PubMed]
- Wu G, Feng X, Stein L. A human functional protein interaction network and its application to cancer data analysis. Genome Biol 2010;11:R53. [Crossref] [PubMed]
- Hoelzinger DB, Demuth T, Berens ME. Autocrine factors that sustain glioma invasion and paracrine biology in the brain microenvironment. J Natl Cancer Inst 2007;99:1583-93. [Crossref] [PubMed]
- Barar J. Targeting tumor microenvironment: the key role of immune system. Bioimpacts 2012;2:1-3. [PubMed]
- Catalona WJ, Mann R, Nime F, et al. Identification of complement-receptor lymphocytes (B cells) in lymph nodes and tumor infiltrates. J Urol 1975;114:915-21. [Crossref] [PubMed]
- Zitvogel L, Apetoh L, Ghiringhelli F, et al. The anticancer immune response: indispensable for therapeutic success? J Clin Invest 2008;118:1991-2001. [Crossref] [PubMed]
- Mariathasan S, Turley SJ, Nickles D, et al. TGFbeta attenuates tumour response to PD-L1 blockade by contributing to exclusion of T cells. Nature 2018;554:544-8. [Crossref] [PubMed]
- Petillo D, Kort EJ, Anema J, et al. MicroRNA profiling of human kidney cancer subtypes. Int J Oncol 2009;35:109-14. [Crossref] [PubMed]
- Muys BR, Sousa JF, Placa JR, et al. miR-450a Acts as a Tumor Suppressor in Ovarian Cancer by Regulating Energy Metabolism. Cancer Res 2019;79:3294-305. [Crossref] [PubMed]
- Shi Y, Gao X, Hu Q, et al. PIK3C2A is a gene-specific target of microRNA-518a-5p in imatinib mesylate-resistant gastrointestinal stromal tumor. Lab Invest 2016;96:652-60. [Crossref] [PubMed]
- Rubie C, Kruse B, Frick VO, et al. Chemokine receptor CCR6 expression is regulated by miR-518a-5p in colorectal cancer cells. J Transl Med 2014;12:48. [Crossref] [PubMed]
- Shao DD, Xue W, Krall EB, et al. KRAS and YAP1 converge to regulate EMT and tumor survival. Cell 2014;158:171-84. [Crossref] [PubMed]
- Salmena L, Carracedo A, Pandolfi PP. Tenets of PTEN tumor suppression. Cell 2008;133:403-14. [Crossref] [PubMed]
- Wang S, Gao J, Lei Q, et al. Prostate-specific deletion of the murine Pten tumor suppressor gene leads to metastatic prostate cancer. Cancer Cell 2003;4:209-21. [Crossref] [PubMed]
- Sansal I, Sellers WR. The biology and clinical relevance of the PTEN tumor suppressor pathway. J Clin Oncol 2004;22:2954-63. [Crossref] [PubMed]
- Gao X, Chen Y, Chen M, et al. Identification of key candidate genes and biological pathways in bladder cancer. PeerJ 2018;6:e6036. [Crossref] [PubMed]
- Peng M, Deng J, Zhou S, et al. Dual Inhibition of Pirarubicin-Induced AKT and ERK Activations by Phenformin Sensitively Suppresses Bladder Cancer Growth. Front Pharmacol 2019;10:1159. [Crossref] [PubMed]
- Huang Y, Zhou S, He C, et al. Phenformin alone or combined with gefitinib inhibits bladder cancer via AMPK and EGFR pathways. Cancer Commun (Lond) 2018;38:50. [Crossref] [PubMed]
- van Hooren L, Sandin LC, Moskalev I, et al. Local checkpoint inhibition of CTLA-4 as a monotherapy or in combination with anti-PD1 prevents the growth of murine bladder cancer. Eur J Immunol 2017;47:385-93. [Crossref] [PubMed]
- Jiang X, Wang J, Deng X, et al. Role of the tumor microenvironment in PD-L1/PD-1-mediated tumor immune escape. Mol Cancer 2019;18:10. [Crossref] [PubMed]
- Zhang S, Liu Y, Liu Z, et al. Transcriptome profiling of a multiple recurrent muscle-invasive urothelial carcinoma of the bladder by deep sequencing. PLoS One 2014;9:e91466. [Crossref] [PubMed]
- Rosenberg JE, Hoffman-Censits J, Powles T, et al. Atezolizumab in patients with locally advanced and metastatic urothelial carcinoma who have progressed following treatment with platinum-based chemotherapy: a single-arm, multicentre, phase 2 trial. Lancet 2016;387:1909-20. [Crossref] [PubMed]
- Mlecnik B, Bindea G, Angell HK, et al. Integrative Analyses of Colorectal Cancer Show Immunoscore Is a Stronger Predictor of Patient Survival Than Microsatellite Instability. Immunity 2016;44:698-711. [Crossref] [PubMed]
- Bingle L, Brown NJ, Lewis CE. The role of tumour-associated macrophages in tumour progression: implications for new anticancer therapies. J Pathol 2002;196:254-65. [Crossref] [PubMed]
- Ou Z, Wang Y, Liu L, et al. Tumor microenvironment B cells increase bladder cancer metastasis via modulation of the IL-8/androgen receptor (AR)/MMPs signals. Oncotarget 2015;6:26065-78. [Crossref] [PubMed]