C3, C3AR1, HLA-DRA, and HLA-E as potential prognostic biomarkers for renal clear cell carcinoma
Introduction
Kidney renal clear cell carcinoma (KIRC) is a malignant tumor originating from the renal epithelium and accounts for approximately 75% of renal tumors (1). Its prognosis is worse than that of other common types of renal cell carcinoma (RCC), such as kidney renal papillary cell carcinoma, and kidney chromophobe (2). The rate of detection with early screening has improved with advances in technology, but about 30% of patients with KIRC are still found to have metastasis at initial diagnosis (3), and 30–35% of patients undergoing surgical treatment eventually develop distant metastasis (4). Although the pathogenesis of KIRC has been extensively studied and many “omics” studies have explored various causes and potential mechanisms for the formation and development of renal cancer, the specific etiology is still unknown, the incidence and mortality of KIRC have remained high over the past decades, and effective diagnostic and prognostic biomarkers are still lacking (5,6). Therefore, finding reliable biomarkers for the early detection and prediction of KIRC is an urgent task.
To seek potential, reliable biomarkers for KIRC, we analyzed transcriptome data from The Cancer Genome Atlas (TCGA) and GSE15641 from the Gene Expression Omnibus (GEO) database. A total of 910 different genes were screened by Gene Ontology (GO) and Kyoto Encyclopedia of Genes and Genomes (KEGG) pathway analyses using “clusterProfiler”, a package of R software (7). Hub genes were screened through the Search Tool for the Retrieval of Interacting Genes (STRING), cytoHubba, and Molecular Complex Detection (MCODE). Subsequently, we selected some interesting hub genes (IHGs) and used Oncomine and The Human Protein Atlas (HPA) databases to analyze their expression as external validation. The prognostic value of these potential biomarkers was analyzed by Kaplan-Meier survival analysis and further verified by the Gene Expression Profiling Interactive Analysis (GEPIA) database; the correlation between gene expression and clinical characteristics and the independent prognostic performance of these potential biomarkers were also fully validated through subsequent analysis. We present the following article in accordance with the MDAR reporting checklist (available at http://dx.doi.org/10.21037/tau-20-699).
Methods
Data acquisition and differential expression analysis
GSE15641 data were obtained from the GEO database (https://www.ncbi.nlm.nih.gov/geo/), comprising 32 tumor samples and 23 normal tissue samples (Platform: GPL96/HG-U133A Affymetrix Human Genome U133A Array) (8). Gene expression data (count) of KIRC patients were downloaded from the TCGA database’s (https://portal.gdc.cancer.gov/) KIRC dataset, comprising 72 normal tissue samples and 539 tumor samples. RNA-sequencing data of 33 types of tumors from the TCGA, which were normalized and transformed into transcripts per kilobase million (TPM) values, were downloaded from the UCSC Xena browser (GDC hub: https://gdc.xenahubs.net). TPM data can make samples more comparable and improve the credibility of the analysis results (9-11). Detailed clinical information of KIRC patients was also obtained from the TCGA data portal (https://portal.gdc.cancer.gov/). The study was conducted in accordance with the Declaration of Helsinki (as revised in 2013). Because the data were obtained from public databases and the retrospective nature of the research, the requirement for informed consent was waived. The code could be obtained after contacting the corresponding author.
To screen for differentially expressed genes (DEGs) in the GSE15641 dataset, GEO2R was used for the analysis (12) with the following screening criteria: adj P<0.05 and |log fold-change| (|log FC|) >2. The edgeR package was used to analyze the gene expression data (count) of the TCGA data with the following cutoff criteria: adj P<0.05 and |log FC| >2 as the (13,14). Subsequently, venny2.1.0 (http://bioinfogp.cnb.csic.es/tools/venny/index.html) was used to identify overlapping DEGs (ODEGs) in the two groups, which were used for further analysis. Upregulated and downregulated genes were downloaded.
GO and KEGG pathway analyses, and construction of the protein-protein interaction (PPI) network
The R Package “clusterProfiler” was used for the GO and KEGG analyses (7). A P value <0.05 was considered a statistically significant result. The results were visualized with a bubble diagram and a circle diagram. The interaction between proteins is important for understanding the metabolic and molecular mechanisms of tumors, so we used the STRING database (https://string-db.org/), which collects related information to predict protein interactions (15). We imported the names of the ODEGs into STRING and set an interaction score of 0.9 points to create a PPI network. Next, we used Cytoscape v3.7.2 to further analyze the data (16), and MCODE was also performed (17). The results were obtained by analyzing with a parameter degree cutoff of 2, a node score cutoff of 0.2, a k-core of 2, and a maximum depth of 100. Meanwhile, cytoHubba was applied to search the hub genes with degree as the evaluation method (18). Finally, we selected some IHGs (C3, C3AR1, HLA-DRA, and HLA-E) from the results of overlapping genes between MCODE and cytoHubba for further analysis and verification.
Verification of gene expression and the prognostic value of IHGs
The Oncomine and HPA databases were used as external validation cohorts to validate the expression of the IHGs. Oncomine, which is an integrated data-mining platform based on the gene chip (https://www.oncomine.org/resource/login.html) (19), was used with the following filter conditions: cancer type, kidney cancer; data type, mRNA; analysis type, cancer vs. normal analysis; threshold setting, P<1e–04, FC >2, gene rank = top 10%. Images of immunohistochemical staining were obtained from the HPA (https://www.proteinatlas.org/). Meanwhile, we also paired the cancer tissue Transcriptome data (TPM) of KIRC from TCGA with normal tissues to evaluate the expression levels of the IHGs. The Wilcoxon test was performed with P<0.05 as the cutoff value.
In addition, we selected KIRC patients from TCGA cohort with complete clinical information and transcriptome data to be analyzed using the Kaplan-Meier method, in order to evaluate the prognostic value of the putative marker genes. The medium expression level was set as the grouping criterion of the high-expression and low-expression groups, and a log-rank P value <0.05 indicated that the difference between the two groups was significant. We used the GEPIA database (http://gepia.cancer-pku.cn/index.html) (20) to verify our results.
Clinical correlation and independent prognostic analysis
The clinical information of KIRC patients from TCGA was combined with gene expression data (TPM) according to their TCGA IDs. Patients with incomplete clinical information were excluded from the analysis. The Wilcoxon and Kruskal-Wallis tests were performed to determine whether the genes significantly correlated with age, gender, tumor grade, American Joint Committee on Cancer (AJCC) stage, T stage, N stage, and M stage. P<0.05 was taken as the critical value. Univariate and multivariate Cox regression analyses were also performed to determine whether the genes could be independent prognostic factors for KIRC, with P<0.05 being taken as the critical value.
Relationship between gene expression and Immune cell infiltration
Tumor Immune Estimation Resource (TIMER) is a comprehensive database (https://cistrome.shinyapps.io/timer/) that includes 10,897 samples from TCGA for estimating the extent of immune cell infiltration (21). We analyzed the correlation between the expression of IHGs and the markers of tumor-infiltrating immune cells, including B cells, CD8+ T cells, CD4+ T cells, monocytes, neutrophils, and dendritic cells. These markers have been cited in previous studies (22,23). Besides, TIMER database was also used to evaluate the correlation of these IHGs after the tumor purity was corrected. P<0.05 was taken as the critical value.
Relationship between potential KIRC biomarkers and immune checkpoints
Immunotherapy is currently one of the most promising tumor treatments, and immune-checkpoint blocking, as an immunotherapy strategy, has shown significant efficacy in the treatment of a range of cancers (24). To indicate the potential contribution of our selected biomarkers in immunotherapy, we evaluated the association between them and some effective immune checkpoints summarized from a previous study (25). Correlation coefficient and P values were calculated by Spearman’s correlation analysis. Furthermore, we selected the top two groups of genes with the highest positive correlation coefficient in KIRC and the one group of genes with a negative correlation coefficient in KIRC, and then calculated the correlation coefficient and P values again in all 33 TCGA cancers by Pearson’s correlation analysis [in a fashion similar to that of Huang et al. (26)] to further analyze our conclusions.
Gene set enrichment analysis (GSEA) of IHGs
GSEA is a threshold-free method of evaluating all genes based on their differential expression rank, or other scores, without prior gene filtering (27). To further explore the biological function of the potential biomarkers we selected, we performed this analysis with GSEA4.0.3 software from the Broad Institute (https://www.gsea-msigdb.org/) and used the annotated gene set of “c2.cp.kegg.v6.2.symbols.gmt”, which was downloaded from MSigDB. After 1,000 permutation steps, a gene set with a P value <0.05 was considered to be significantly enriched.
Statistical analysis
Mann-Whitney U tests (also called the Wilcoxon rank-sum test) were used to conduct difference comparisons of two groups and Kruskal-Wallis tests were used for the comparisons of three or more groups. Correlations coefficients between the expression level of potential biomarkers and the expression level of several immune checkpoints were computed by Spearman and distance correlation analyses. The prognostic value of these potential biomarkers was showed by the survival curves which were generated through the Kaplan-Meier method and the log-rank (Mantel-Cox) test was performed to identify the statistical significance of differences. The univariate Cox regression model was used to calculate the hazard ratios (HRs) for these potential biomarkers and the multivariable Cox regression model was used to identify independent prognostic factors using the survminer package. All statistical P value were two-side and P<0.05 means the result has statistically significance. All statistical analyses were conducted through R 3.6.2 software.
Results
Identification of ODEGs
The study flowchart is shown in Figure 1: 1,575 upregulated and 468 downregulated genes were selected from GSE15641, and 10,455 upregulated, and 3,177 downregulated genes were screened from TCGA. From this, we obtained ODEGs, comprising 671 upregulated and 239 downregulated genes (Figure 2A,B).
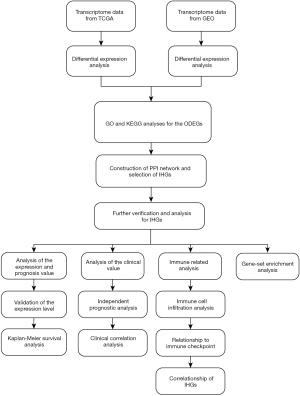
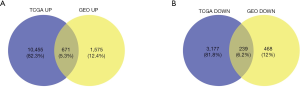
Construction of enrichment analysis, PPI network, and screening of hub genes
By analyzing the GO function, we found that the ODEGs were enriched in some cellular components (CCs) such as collagen-containing extracellular matrix (ECM), endoplasmic reticulum lumen, and major histocompatibility complex protein complex. In terms of biological processes (BPs), the ODEGs were enriched in functions of leukocyte migration, extracellular structure organization, and ECM organization. In terms of molecular function (MF), peptide antigen binding, glycosaminoglycan binding, and the ECM structural constituent were found in the first enrichment classes (Figure 3A). All information is presented in Table 1. Through KEGG pathway analysis, we found the ODEGs mainly occurred in the HIF-1 signaling and PPAR signaling pathways, but were present in another six pathways (Figure 3B). The eight pathways, each with P<0.05, are shown in Table 2.
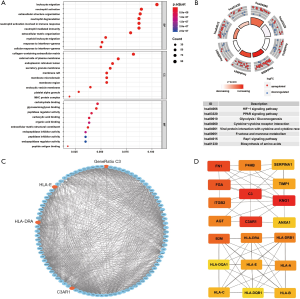
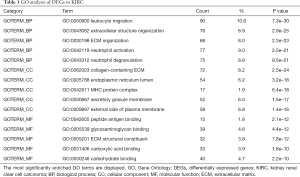
Full table
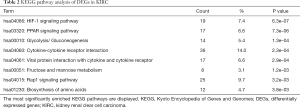
Full table
The PPI network was constructed by STRING, and the data were imported into Cytoscape. One module of hub genes was selected by MCODE (Figure 3C), and 20 Hub genes were identified by cytoHubba (Figure 3D). We comprehensively analyzed the results of these two methods and screened four IHGs (C3, C3AR1, HLA-DRA, and HLA-E) from the overlapping genes from MCODE and cytoHubba for further analysis.
Differential expression of IHGs and its influence on the prognosis of patients
Meta-analysis in Oncomine was performed to verify the differential expression of the four IHGs, and the result showed that all of them were significantly upregulated in KIRC tissues (8,28-31) (Figure 4A). The paired analysis between cancer and normal tissues also showed that these IHGs were significantly highly expressed in KIRC (P<0.001) (Figure 4B). Immunohistochemical staining showed that C3 presented as high intensity, HLA-DRA and HLA-E as medium intensity, and C3AR1 as low intensity in tumor tissue (Figure 4C).
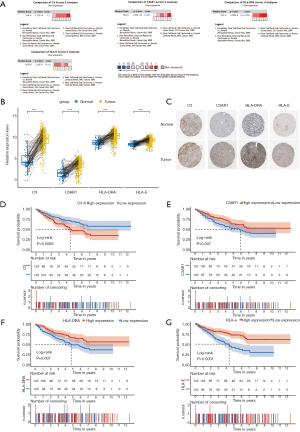
Kaplan-Meier survival analysis was performed for the 246 KIRC patients from TCGA with complete clinical and transcriptome data. The survival curves plotted based on the overall survival (OS) data indicated that all four IHGs could significantly affect the prognosis of patients (Figure 4D,E,F,G); that is, the high expression of C3 could increase the risk of a poor prognosis (log-rank P=0.0083), while the high expression of C3AR1, HLA-DRA, and HLA-E could increase the possibility of better prognosis (C3AR1: log-rank P=0.047; HLA-DRA: log-rank P=0.007; HLA-E: log-rank P<0.0001). We used the GEPIA database to verify the role of these genes in KIRC patients and obtained a similar result (Figure 5).
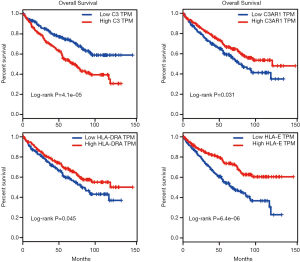
Association of potential biomarkers with clinical characteristics of KIRC patients
TPM data of KIRC patients with complete clinical and transcriptome information were used to perform the clinical correlation analysis (Figure 6). The results showed that C3 expression showed a higher trend in patients with higher degree of tumor malignancy (grade: P<0.001; stage: P<0.001) and the difference in TNM-stage expression level was also statistically significant (T-stage: P<0.001; N-stage: P<0.05; M-stage: P<0.05). Thus, the high expression level of C3 may indicate the presence of tumor spread. Meanwhile, the expression levels of C3AR1, HLA-E, and HLA-DRA in different tumor grades were also significantly different (C3AR1: P<0.05; HLA-E: P<0.01; HLA-DRA: P<0.05).
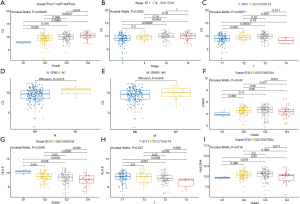
Univariate Cox regression analysis also showed that all four genes could affect the prognosis of KIRC patients (P<0.05) and further multivariate Cox regression identified C3AR1 (P<0.01), HLA-DRA (P<0.001), and HLA-E (P<0.001) as independent prognostic factors for KIRC patients (Table 3).
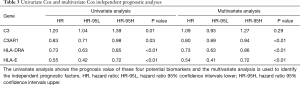
Full table
Expression of each IHG and immune cell infiltration of KIRC
Tumor-infiltrating lymphocytes are an independent marker of sentinel lymph node status and cancer survival. Therefore, we investigated the association between the expression of the IHGs and immune cell infiltration in KIRC. We found that the expression level of C3 had a significant correlation with infiltrating B cells, macrophages, neutrophils, and dendritic cells (P<0.05). C3AR1, HLA-DRA, and HLA-E had significant correlations with purity, B cells, CD8+ T cells, CD4+ T cells, monocytes, neutrophils, and dendritic cells (P<0.05) (Figure 7). These results strongly suggest that these four genes play a specific role in immune cell infiltration of KIRC, which brings new hope for immunotherapy. After tumor purity was corrected by the TIMER database, we found these genes had close relationships, with C3AR1 and HLA-DRA being the closest genes (Partial cor =0.769, P=2.42e–91) (Figure 8). These results will assist in further understanding the function and mechanisms of these genes in oncogenesis.
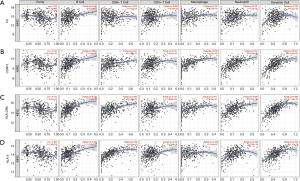
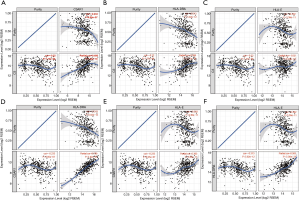
Special role of potential biomarkers in the immunotherapy of KIRC
Spearman’s correlation analysis showed the expression levels of C3AR1, HLA-DRA, and HLA-E were positively correlated with immune-checkpoint genes, and the correlation was statistically significant (P<0.01). Only C3 had a negative correlation with CD274 (PD-L1) (P<0.01) (Figure 9A). The relationship between C3 and PD-L1 was significant in KIRC, which was not shown in other types of RCC (Figure 9B). HLA-DRA and PDCD1LG2 had the highest correlation coefficient (cor =0.74) followed by C3AR1 and PDCD1LG2 (cor =0.73). The pan-cancer analysis in RCC indicated that both C3AR1 and HLA-DRA had a strong positive correlation with PDCD1LG2 in three common types of RCC (Figure 9C,D).
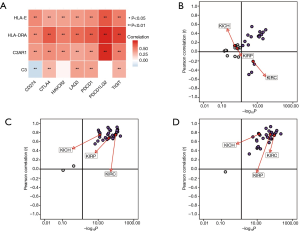
GSEA and the biological function of hub genes in KIRC
Through GSEA analysis, a powerful analytical method for identifying sets of genes regulated differently in one direction (32), we found all four genes were enriched in cytokine-cytokine receptor interaction, chemokine signaling pathway, and some immune-related pathways. C3AR1 was enriched in the cancer pathway. C3, HLA-DRA, and HLA-E were significantly enriched in the JAK-STAT signaling pathway and natural killer cell-mediated cytotoxicity (Figure 10).
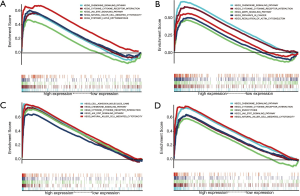
Discussion
Tumor markers have a wide range of applications in predicting the occurrence and development of tumors, along with the prognosis of patients. Our study identified four genes as potential tumor markers of KIRC. Previous studies have used bioinformatics methods to search for potential RCC markers (5), but we used more validation methods to prove the reliability of our conclusions on multiple dimensions. Moreover, we deeply analyzed the relationship between potential biomarkers and immune checkpoints, and then selected several key groups for further validation at the pan-cancer level to explore their unique role in KIRC immunotherapy. Studies pay attention to the role of these four biomarkers in KIRC patients were rare, especially for C3AR1 and HLA-DRA. Our study systematically and comprehensively analyzed their potential role in the clinical characters, prognosis and immunotherapy of KIRC patients, providing strong evidence and research direction for further research.
Complement component C3 plays a central role in the activation of the complement system. Both classical and alternative complement activation pathways are needed to activate it. If lacking, the immune system may be damaged (33). The relationship between C3 and tumorigenesis is a research hotspot. Zha et al. suggested tumor cell-derived C3 may be an effective target for tumor immunotherapy (34). Abnormal expression of C3 is likely to harm cancer patients, and although the role of C3 in colorectal cancer (35) and liver cancer (36) has been explored, research on C3 in KIRC is still lacking. Our study deeply and comprehensively analyzed the relationship between C3 and the prognosis of KIRC patients. We found that C3 is a significant indicator for these patients. Upregulation of its expression level may reveal the occurrence and development of tumors. We also conducted a pan-cancer analysis of the relationship between C3 and CD274 within the scope of RCC and found they both have a unique negative correlation in KIRC, which suggests that C3 may be involved in the regulatory pathway of PD-L1 (coding gene: CD274). This unique correlation also revealed C3’s potential as an immune target for KIRC. The GO and KEGG analyses showed that C3 is mainly enriched in biological functions or pathways related to immunity, inflammation, and infection.
C3AR1 is an orphan G protein-coupled receptor for C3a, which is decomposed by C3. C3 and C3AR1 are closely related and play important roles in many BPs, such as the C3-C3a-C3AR1 pathway (37) and the formation of intestinal organoids (38). However, there has not been a study based on the relationship between C3AR1 and KIRC, and ours is the first to propose that C3AR1 is a potential biomarker for KIRC. After a comprehensive analysis, we found that C3AR1 is a significant protective factor and an independent risk factor for KIRC patients. Meanwhile, C3AR1 showed a significant correlation with various immune-checkpoints, and the strongest correlation was with PDCD1LG2, a correlation that exists in other types of RCC in particular and in most other types of cancers. In addition, immune cell infiltration analysis found that C3AR1 was most correlated with macrophages in KIRC patients, which provides ideas and directions for precise immunotherapy in KIRC patients.
Compared with other HLA genes, the reported polymorphism of the HLA-DRA gene is limited (39), and the important role of this gene in the occurrence and development of cervical cancer (40), rectal cancer (41), and ductal cancer (42) has been studied. However, the relationship between this gene and KIRC has not been reported, and we found it acted as a protective, independent prognostic factor in KIRC patients. Iwahashi et al. found valproic acid combined with gemcitabine could regulate the expression level of HLA-DRA, and the differentiation or apoptosis of tumor cells (43). Our study found that HLA-DRA was closely correlated with PDCD1LG2, and our pan-cancer analysis found that this relationship existed in most tumor types, which may partly explain the regulatory mechanism of HLA-DRA in the immunotherapy process, and further reveals its great potential as an immunotherapy target.
HLA-E is a non-classical class HLA-I molecule associated with tumor immune evasion. Studies have shown that HLA-E overexpression often occurs in RCC and is related to immunogenicity reduction (44). There is a positive correlation between HLA-E expression and a better Fuhrmann score (45), which is consistent with our finding that the high expression of HLA-E improved OS in KIRC patients. Our study further demonstrated that HLA-E is a protective, independent prognostic factor for KIRC patients, and the higher the degree of tumor malignancy, the lower the expression level. Similar to HLA-DRA and C3AR1, HLA-E is also closely related to CD274, PDCD1LG2, and TIGIT, and its unique contribution in the process of KIRC immunotherapy deserves more attention.
There are still some limitations and deficiencies of this study. Firstly, it was a purely bioinformatics study, and its scientific hypothesis has not been verified by biological experiments. Secondly, although database data were used, the sample size was still limited. In the future, we will conduct corresponding in vivo and in vitro experiments to validate the specific functions of the selected tumor markers and continue to explore the potential carcinogenic mechanism of hub genes in combination with multi-omics analysis.
Conclusions
We analyzed KIRC transcriptome data through a comprehensive bioinformatics approach with the help of TCGA and GEO. Our study identified C3, C3AR1, HLA-DRA, and HLA-E as potential tumor markers of KIRC, and their expression had a close correlation with tumor immune cell infiltrates. Moreover, C3AR1, HLA-DRA, and HLA-E could be independent prognostic factors of KIRC. C3 and CD274 showed special correlations in KIRC compared with other types of RCC, which may provide new directions for the immunotherapy of KIRC. The results of this research will have a positive effect on the early detection, early treatment, and early cure of KIRC, thus improving the prognosis of KIRC patients.
Acknowledgments
We thank the AME Editing Service (http://editing.amegroups.com/) for improving our paper.
Funding: This work was supported by the National Natural Science Foundations of China under Grant 81772713, 81472411, 81981260351, 81972378; Taishan Scholar Program of Shandong Province under Grant tsqn20161077; Natural Science Foundation of Shandong Province under Grant ZR2016HQ18; Key Research and Development Program of Shandong Province under Grant 2018GSF118197.
Footnote
Reporting Checklist: The authors have completed the MDAR reporting checklist. Available at http://dx.doi.org/10.21037/tau-20-699
Conflicts of Interest: All authors have completed the ICMJE uniform disclosure form (available at http://dx.doi.org/10.21037/tau-20-699). The authors have no conflicts of interest to declare.
Ethical Statement: The authors are accountable for all aspects of the work in ensuring that questions related to the accuracy or integrity of any part of the work are appropriately investigated and resolved. The study was conducted in accordance with the Declaration of Helsinki (as revised in 2013). Because the data were obtained from public databases and the retrospective nature of the research, the requirement for informed consent was waived.
Open Access Statement: This is an Open Access article distributed in accordance with the Creative Commons Attribution-NonCommercial-NoDerivs 4.0 International License (CC BY-NC-ND 4.0), which permits the non-commercial replication and distribution of the article with the strict proviso that no changes or edits are made and the original work is properly cited (including links to both the formal publication through the relevant DOI and the license). See: https://creativecommons.org/licenses/by-nc-nd/4.0/.
References
- Hsieh JJ, Purdue MP, Signoretti S, et al. Renal cell carcinoma. Nat Rev Dis Primers 2017;3:17009. [Crossref] [PubMed]
- Ljungberg B, Albiges L, Abu-Ghanem Y, et al. European Association of Urology Guidelines on renal cell carcinoma: the 2019 update. Eur Urol 2019;75:799-810. [Crossref] [PubMed]
- Gupta K, Miller JD, Li JZ, et al. Epidemiologic and socioeconomic burden of metastatic renal cell carcinoma (mRCC): a literature review. Cancer Treat Rev 2008;34:193-205. [Crossref] [PubMed]
- Porta C, Cosmai L, Leibovich BC, et al. The adjuvant treatment of kidney cancer: a multidisciplinary outlook. Nat Rev Nephrol 2019;15:423-33. [Crossref] [PubMed]
- Song E, Song W, Ren M, et al. Identification of potential crucial genes associated with carcinogenesis of clear cell renal cell carcinoma. J Cell Biochem 2018;119:5163-74. [Crossref] [PubMed]
- Yang JF, Shi SN, Xu WH, et al. Screening, identification and validation of CCND1 and PECAM1/CD31 for predicting prognosis in renal cell carcinoma patients. Aging (Albany NY) 2019;11:12057-79. [Crossref] [PubMed]
- Yu G, Wang LG, Han Y, et al. clusterProfiler: an R package for comparing biological themes among gene clusters. OMICS 2012;16:284-7. [Crossref] [PubMed]
- Jones J, Otu H, Spentzos D, et al. Gene signatures of progression and metastasis in renal cell cancer. Clin Cancer Res 2005;11:5730-9. [Crossref] [PubMed]
- Vivian J, Rao AA, Nothaft FA, et al. Toil enables reproducible, open source, big biomedical data analyses. Nat Biotechnol 2017;35:314-6. [Crossref] [PubMed]
- Wagner GP, Kin K, Lynch VJ. Measurement of mRNA abundance using RNA-seq data: RPKM measure is inconsistent among samples. Theory Biosci 2012;131:281-5. [Crossref] [PubMed]
- Zeng D, Li M, Zhou R, et al. Tumor microenvironment characterization in gastric cancer identifies prognostic and immunotherapeutically relevant gene signatures. Cancer Immunol Res 2019;7:737-50. [Crossref] [PubMed]
- Barrett T, Wilhite SE, Ledoux P, et al. NCBI GEO: archive for functional genomics data sets--update. Nucleic Acids Res 2013;41:D991-5. [Crossref] [PubMed]
- McCarthy DJ, Chen Y, Smyth GK. Differential expression analysis of multifactor RNA-Seq experiments with respect to biological variation. Nucleic Acids Res 2012;40:4288-97. [Crossref] [PubMed]
- Robinson MD, McCarthy DJ, Smyth GK. edgeR: a Bioconductor package for differential expression analysis of digital gene expression data. Bioinformatics 2010;26:139-40. [Crossref] [PubMed]
- Szklarczyk D, Franceschini A, Wyder S, et al. STRING v10: protein-protein interaction networks, integrated over the tree of life. Nucleic Acids Res 2015;43:D447-52. [Crossref] [PubMed]
- Shannon P, Markiel A, Ozier O, et al. Cytoscape: a software environment for integrated models of biomolecular interaction networks. Genome Res 2003;13:2498-504. [Crossref] [PubMed]
- Bader GD, Hogue CW. An automated method for finding molecular complexes in large protein interaction networks. BMC Bioinformatics 2003;4:2. [Crossref] [PubMed]
- Chin CH, Chen SH, Wu HH, et al. cytoHubba: identifying hub objects and sub-networks from complex interactome. BMC Syst Biol 2014;8 Suppl 4:S11. [Crossref] [PubMed]
- Rhodes DR, Yu J, Shanker K, et al. ONCOMINE: a cancer microarray database and integrated data-mining platform. Neoplasia 2004;6:1-6. [Crossref] [PubMed]
- Tang Z, Li C, Kang B, et al. GEPIA: a web server for cancer and normal gene expression profiling and interactive analyses. Nucleic Acids Res 2017;45:W98-102. [Crossref] [PubMed]
- Li T, Fan J, Wang B, et al. TIMER: a web server for comprehensive analysis of tumor-infiltrating immune cells. Cancer Res 2017;77:e108-10. [Crossref] [PubMed]
- Danaher P, Warren S, Dennis L, et al. Gene expression markers of Tumor Infiltrating Leukocytes. J Immunother Cancer 2017;5:18. [Crossref] [PubMed]
- Siemers NO, Holloway JL, Chang H, et al. Genome-wide association analysis identifies genetic correlates of immune infiltrates in solid tumors. PLoS One 2017;12:e0179726. [Crossref] [PubMed]
- Postow MA, Sidlow R, Hellmann MD. Immune-related adverse events associated with immune checkpoint blockade. N Engl J Med 2018;378:158-68. [Crossref] [PubMed]
- Mariathasan S, Turley SJ, Nickles D, et al. TGFbeta attenuates tumour response to PD-L1 blockade by contributing to exclusion of T cells. Nature 2018;554:544-8. [Crossref] [PubMed]
- Huang H, Weng H, Zhou K, et al. Histone H3 trimethylation at lysine 36 guides m(6)A RNA modification co-transcriptionally. Nature 2019;567:414-9. [Crossref] [PubMed]
- Reimand J, Isserlin R, Voisin V, et al. Pathway enrichment analysis and visualization of omics data using g:Profiler, GSEA, Cytoscape and EnrichmentMap. Nat Protoc 2019;14:482-517. [Crossref] [PubMed]
- Yusenko MV, Kuiper RP, Boethe T, et al. High-resolution DNA copy number and gene expression analyses distinguish chromophobe renal cell carcinomas and renal oncocytomas. BMC Cancer 2009;9:152. [Crossref] [PubMed]
- Gumz ML, Zou H, Kreinest PA, et al. Secreted frizzled-related protein 1 loss contributes to tumor phenotype of clear cell renal cell carcinoma. Clin Cancer Res 2007;13:4740-9. [Crossref] [PubMed]
- Beroukhim R, Brunet JP, Di Napoli A, et al. Patterns of gene expression and copy-number alterations in von-hippel lindau disease-associated and sporadic clear cell carcinoma of the kidney. Cancer Res 2009;69:4674-81. [Crossref] [PubMed]
- Lenburg ME, Liou LS, Gerry NP, et al. Previously unidentified changes in renal cell carcinoma gene expression identified by parametric analysis of microarray data. BMC Cancer 2003;3:31. [Crossref] [PubMed]
- Saxena V, Orgill D, Kohane I. Absolute enrichment: gene set enrichment analysis for homeostatic systems. Nucleic Acids Res 2006;34:e151. [Crossref] [PubMed]
- Pio R, Corrales L, Lambris JD. The role of complement in tumor growth. Adv Exp Med Biol 2014;772:229-62. [Crossref] [PubMed]
- Zha H, Wang X, Zhu Y, et al. Intracellular activation of complement C3 leads to PD-L1 antibody treatment resistance by modulating tumor-associated macrophages. Cancer Immunol Res 2019;7:193-207. [Crossref] [PubMed]
- Qiu Y, Patwa TH, Xu L, et al. Plasma glycoprotein profiling for colorectal cancer biomarker identification by lectin glycoarray and lectin blot. J Proteome Res 2008;7:1693-703. [Crossref] [PubMed]
- Goldman R, Ressom HW, Abdel-Hamid M, et al. Candidate markers for the detection of hepatocellular carcinoma in low-molecular weight fraction of serum. Carcinogenesis 2007;28:2149-53. [Crossref] [PubMed]
- Litvinchuk A, Wan YW, Swartzlander DB, et al. Complement C3aR inactivation attenuates Tau pathology and reverses an immune network deregulated in Tauopathy models and Alzheimer's disease. Neuron 2018;100:1337-53.e5. [Crossref] [PubMed]
- Matsumoto N, Satyam A, Geha M, et al. C3a enhances the formation of intestinal organoids through C3aR1. Front Immunol 2017;8:1046. [Crossref] [PubMed]
- Matern BM, Olieslagers TI, Voorter CEM, et al. Insights into the polymorphism in HLA-DRA and its evolutionary relationship with HLA haplotypes. HLA 2020;95:117-27. [Crossref] [PubMed]
- Samuels S, Spaans VM, Osse M, et al. Human leukocyte antigen-DR expression is significantly related to an increased disease-free and disease-specific survival in patients with cervical adenocarcinoma. Int J Gynecol Cancer 2016;26:1503-9. [Crossref] [PubMed]
- Agostini M, Janssen KP, Kim IJ, et al. An integrative approach for the identification of prognostic and predictive biomarkers in rectal cancer. Oncotarget 2015;6:32561-74. [Crossref] [PubMed]
- Song G, He L, Yang X, et al. Identification of aberrant gene expression during breast ductal carcinoma in situ progression to invasive ductal carcinoma. J Int Med Res 2020;48:300060518815364. [Crossref] [PubMed]
- Iwahashi S, Shimada M, Utsunomiya T, et al. Histone deacetylase inhibitor enhances the anti-tumor effect of gemcitabine: a special reference to gene-expression microarray analysis. Oncol Rep 2011;26:1057-62. [PubMed]
- Seliger B, Jasinski-Bergner S, Quandt D, et al. HLA-E expression and its clinical relevance in human renal cell carcinoma. Oncotarget 2016;7:67360-72. [Crossref] [PubMed]
- Kren L, Valkovsky I, Dolezel J, et al. HLA-G and HLA-E specific mRNAs connote opposite prognostic significance in renal cell carcinoma. Diagn Pathol 2012;7:58. [Crossref] [PubMed]